Wearable electrochemical sensors for real-time monitoring in diabetes mellitus and associated complications
Abstract
This comprehensive review underscores the pivotal role wearable electrochemical sensors play in the proactive management and prevention of diabetes mellitus (DM) and its associated complications. Acknowledging the substantial impact of DM on individuals and the urgency for effective monitoring strategies, wearable sensors have emerged as a pragmatic solution. These sensors can detect analytical signals from biofluids, including sweat, tears, saliva, and interstitial fluid (ISF), employing minimally invasive techniques facilitated by technological advancements. The seamless integration of these sensors with computational platforms such as smartphones enhances their practicality for routine use. The review systematically explores diverse methodologies, encompassing both enzymatic and non-enzymatic principles, employed for the surveillance of analytes within biofluids. These foundational principles are meticulously applied to wearable devices, affording point-of-care solutions catering to the detection of individual analytes or simultaneous multiplexed analyte detection. The integration of wireless systems and the incorporation of machine learning algorithms introduce a layer of sophistication, elevating the capability of these sensors for the nuanced monitoring of DM and its complications. Through an in-depth analysis of these advancements, this review describes the significant potential of wearable electrochemical sensors as an essential tool for real-time monitoring and managing DM. The diverse approaches presented underscore the adaptability, versatility, and inherent efficacy of these sensors in addressing the multifaceted challenges intrinsic to DM and its associated complications within academic discourse.
Keywords
INTRODUCTION
The global epidemic of diabetes has become evident with the advent of widespread industrialization and a substantial escalation in obesity rates[1]. According to the International Diabetes Federation, the global prevalence of diabetes mellitus (DM) among individuals aged 20 to 79 was estimated at 537 million in 2021. According to the International Diabetes Federation, this number is expected to grow to 643 million by 2030 and further escalate to 783 million by 2045[2]. DM, an incurable chronic disease, is identified as a metabolic disorder marked by persistent hyperglycemia. Its pivotal characteristic involves as inherent insufficiency in the secretion and/or action of insulin by pancreatic β cells, whether absolute or relative[3,4]. In instances of severe hyperglycemia, common clinical symptoms include increased thirst (polydipsia) and excessive urination (polyuria). In extreme cases, DM can lead to coma. On the other hand, in mild cases of hyperglycemia, patients with diabetes might not show any signs, and if ignored, this situation could result in fatal outcomes[5]. Also, diabetes is intricately associated with and actively contributes to a diverse range of complications, encompassing cerebrovascular disease[6-8], cardiovascular symptoms[9-11], diabetic kidney disease (nephropathy)[12-14], Pancreatogenic diabetes[15-17], and other related conditions[18-21]. Given the substantial health risks associated with DM, it is imperative for individuals to consistently monitor and maintain optimal concentrations of key biomarkers pertinent to diabetes and its associated complications, as outlined in Tables 1 and 2.
Physiological reference range of metabolites and electrolytes in biofluids for individuals in good health (units: mM)
Biofluids | Glucose | Lactate | Uric acid | Sodium | Potassium |
Blood | 3.9~5.6[22,23] | 0.5~1.5[24,25] | 0.2~0.42[26,27] | 135~150[28-30] | 5~6[30-32] |
Sweat | 0.06~0.11[33-35] | 16~30[36,37] | ~0.02[38] | 10~100[28-30] | 4~24[30-32] |
Saliva | 0.03~0.1[38,39] | 0.09~0.13[40] | 0.17~0.23[41] | 8.7~217.3[42,43] | 2.6~18.3[42,43] |
Tear | 0.06~0.3[44] | 1~5[45] | 0.07~0.16[46] | 120~170[47] | 6~42[47] |
ISF | 3.2~9.2[48-50] | 0.5~10[51] | - | 120~154[52,53] | 2.8~5.3[54,55] |
Physiological reference range of metabolites and electrolytes in biofluids for individuals with diabetic chronic complications (units: mM)
As a solution for point-of-care systems in managing DM, blood glucose monitoring has played a critical part, and over the past three decades, with the introduction of continuous glucose monitoring (CGM) devices, tremendous progress has been made toward commercialization[58-60]. CGM technologies leverage electrochemical and optical methods for real-time glucose monitoring. Electrochemical CGMs, prevalent in current use, operate based on enzyme reactions that produce an electrical signal correlating with glucose concentrations. Despite their proven accuracy and capability for real-time monitoring, these devices encounter limitations, including relatively short lifespans, the necessity for regular calibration, potential for skin irritation, and their restriction to monitoring glucose alone. The optical CGM introduces a different approach, utilizing light and fluorescent chemistry, which potentially extends the lifespan of sensors and reduces the need for frequent replacements. However, it faces challenges similar to those of its electrochemical counterparts, such as skin irritation, and is also limited to monitoring glucose exclusively. Notably, users of the optical CGM are required to visit a physician for sensor setup and replacement every three months[61]. Furthermore, ongoing extensive efforts and research endeavors aim to foster the broad acceptance of wearable sensor technology[62]. This involves the implementation of flexible, non-invasive wearable sensors designed for seamless integration into lifestyles of individuals, mitigating the discomfort typically associated with conventional finger prick blood testing methods. Additionally, this technology enables multiplexed sensing capabilities using millimeter-long microneedle arrays (MNAs) and other non-invasive methods, which not only facilitate minimal tissue inflammation and rapid skin recovery but also allow for the simultaneous measurement of multiple analytes[63].
These wearable sensors serve as pivotal tools in preserving optimal health for individuals with DM and preventing associated complications. They enable real-time monitoring of key metabolites, including glucose (a gold standard biomarker for DM)[64-66], lactate (relevant to diabetic kidney disease)[67-69], ketones [associated with diabetic ketoacidosis (DKA) and potential coma][70-72], uric acid (linked to cardiovascular and renal diseases)[73-75], and electrolytes [critical for identifying electrolyte disorders such as sodium (Na+) and potassium (K+)][76-78]. These sensors operate across various human biofluids, encompassing tears[79-82], saliva, blood serum, interstitial fluid (ISF), and sweat[83,84].
Among a number of methods for monitoring biomarkers related to DM, electrochemical analysis has been widely investigated[85]. This strategy, which offers a straightforward and quantitative approach, measures electrochemical signals and converts them directly into concentrations of metabolites and electrolytes. Consequently, it is the most commonly utilized method, generally relying on potentiometric[86-88], amperometric[89-91], and voltametric techniques[92-94]. Furthermore, electrochemical sensors are currently gaining traction due to their miniaturization, low-power consumption instrumentation, and the potential for implementation in wearable devices that enable simple, accurate, sensitive, selective, and low-cost analytical procedures[95,96].
This review offers a comprehensive overview of wearable sensor technology utilizing electrochemical analysis for managing DM and its associated complications. The primary focus is on non-invasive and minimally invasive electrochemical wearable sensors specifically engineered for detecting various metabolites and electrolytes present in human biofluids. The initial section of the review delves into the electrochemical sensing mechanisms applied to monitor biomolecules and electrolytes. This includes discussions on the materials employed, such as enzymes, non-enzyme assays, polymer-based ion-selective membranes (ISM), and the different sensing modes used for wearable electrochemical sensors. Following that, the second section explored wearable electrochemical sensors, elucidating device designs specifically designed for monitoring targeted metabolites and electrolytes in biofluids such as sweat, tears, saliva, and ISF, aimed at enhancing the effectiveness of DM management. The subsequent section of the review focuses on wearable sensor devices for a multiplexed monitoring platform. This involves chemical-electrophysiological hybrid sensing systems, along with multiplexed electrochemical sensors that can simultaneously monitor various biomarkers for effective DM management. Additionally, the review explores machine learning (ML)-based multiplexed analysis methodologies specifically designed for the efficient management of DM and its associated complications [Figure 1].
Figure 1. Schematic illustration of strategic approaches utilizing wearable devices for monitoring diabetes mellitus and its complications. ISF: Interstitial fluid; ECG: electrocardiogram; PPG: photoplethysmography; RNN: recurrent neural networks; CNN: convolutional neural networks; LSTM: long short-term memory; ARIMA: autoregressive integrated moving average; SVM: support vector machines; KNN: k nearest neighbors.
STRATEGIES FOR ELECTROCHEMICAL SENSING
Electrochemical biosensors assume a pivotal role in identifying biomarkers within biofluids, including sweat, saliva, tears, and ISF, facilitating the delineation of risk stages in chronic diseases[97]. Moreover, the real-time monitoring of various DM-related parameters, such as glucose, lactate, uric acid, and electrolyte, during daily life provides valuable data for assessing DM management of an individual[64,78]. The intricacies of biomarker detection necessitate a systematic approach to sensor development, ensuring precision in sensing and translating biomarker research into clinically applicable solutions[98]. There are three different types of electrochemical sensors: potentiometric[86-88]; amperometric[89-91]; voltametric[92-94].
Conventionally, electrochemical sensors comprise three electrodes: the working electrode (WE), reference electrode (RE), and counter electrode (CE)[99]. Voltammetry, particularly cyclic voltammetry, is acknowledged as a traditional electrochemical technique. This approach entails measuring the current within controlled potential conditions. In contrast, amperometric sensors function as apparatuses dedicated to quantifying the current resulting from the oxidation and reduction processes inherent in biochemical reactions. Potentiometric electrochemical sensors are composed of two electrodes: the WE and RE[100]. In potentiometric measurements, these sensors assess the charge accumulation at the WE concerning the RE. These types of electrochemical sensors comprise enzymatic and non-enzymatic sensors, and a wide range of materials has been used in both enzymatic and non-enzymatic electrochemical biosensors such as enzymes, antibodies, aptamers, molecular imprinted polymers (MIPs), and polymer-based ISM.
Enzymatic electrochemical sensing
Enzyme
Enzymes are crucial in constructing wearable electrochemical biosensors. Through simple enzymatic reactions targeting biomarkers such as glucose, lactate, and uric acid, biosensors offer numerous advantages, manifesting in cost-effectiveness, rapid result acquisition, and necessitating minimal sample volume for measurement.
The fundamental concept of an enzyme-based biosensing electrode involves immobilizing enzyme molecules in close proximity to an electrode surface. Among the enzyme families associated with biomarkers for DM and its related complications, glucose oxidase (GOX) is commonly employed for glucose monitoring in DM management[101]. Similarly, lactate oxidase (LOX) and lactate dehydrogenase (LDH) are used in developing lactate biosensors[102], while uricase is employed for uric detection[103]. According to the difference of electron transfer mechanisms, various architectural designs of enzyme-based electrochemical biosensors measuring free electrons emitted from enzymatic reactions are illustrated in Figure 2A-C.
Figure 2. Response mechanisms of enzymatic electrochemical sensors and enzyme immobilization methods. (A) Schematic illustration of the first-generation enzymatic sensor utilizes the concept of electrocatalytic detection; (B) Schematic representation of the second-generation enzymatic sensor incorporates redox mediators; (C) The third-generation enzymatic sensor; (D) Schematic illustration for physical enzyme immobilization of soaking method, drying method, entrapment method, and ionic binding, respectively; (E) Diagram illustrating enzyme immobilization of chemical methods of covalent bonding and crosslinking, respectively.
First-generation electrochemical biosensors utilize enzymes that catalyze reactions involving either the consumption of electroactive reactants (e.g., O2) or the production of electroactive species (e.g., H2O2) [Figure 2A]. The depletion of the target substrate (e.g., glucose, lactate, etc.) or the increase in the product (electrochemically active H2O2) is then monitored to determine the concentration of the target analyte. The current, which is directly proportional to the concentration of biomarkers, arises from the oxidation of H2O2 at the WE. It is important to note that oxygen serves as the physiological electron acceptor in this oxidase-based concept. First-generation biosensors have demonstrated remarkable sensitivity and are distinguished by exceedingly short response times, typically on the order of one second [104]. Nevertheless, the stoichiometric constraints of oxygen and the inherent fluctuations in its levels within biofluids may introduce inaccuracies in this initial conceptualization[105]. Additionally, the exigency of a high potential for directly detecting targeted analytes impedes selectivity toward the desired analyte by inducing the unintended oxidation of untargeted analytes[106].
Second-generation enzyme-based sensors incorporate redox mediators that directly interact with enzymes, addressing the challenge of high potential requirements. These mediators, such as redox dye compounds or transition metal-based compounds, facilitate electron transfer from the active site of the enzyme to the electrode surface at a lower potential than needed for H2O2 oxidation[107,108]. Among the most prevalent and widely recognized mediators in electrochemical biosensing are ferricyanide and ferrocene (Fc). Additionally, other notable mediators include methylene blue, phenazines, methyl violet, alizarin yellow, Prussian blue, thionin, azure A and C, toluidine blue, and inorganic redox ions, all of which find extensive utilization in this field[109]. Additional enhancements are achieved by substituting oxygen with an electron acceptor capable of facilitating electron transfer from the redox center of the enzyme to the electrode. As depicted in Figure 2B, the MEDRED undergoes oxidation at the electrode surface, thereby generating a current signal that correlates with the concentration of detected biomarkers. Here, MEDOX and MEDRED represent the oxidized and reduced forms of the mediator, respectively[85]. This integration improves electron transfer acceleration, mitigates the oxygen effect, and enhances stability, sensitivity, and selectivity of biosensors compared to first-generation oxidase-based models[110]. Careful mediator selection, considering attributes such as solubility and biocompatibility, is crucial for in-vivo applications.
Third-generation enzyme-based sensors utilize either redox or engineered enzymes with modified structures to enable direct electron transfer between the redox center of the enzyme and the electrode surface[111] [Figure 2C]. The absence of mediators in these biosensors is a notable advantage, operating within a potential window closer to the redox potential of the enzyme, resulting in reduced susceptibility to interfering reactions and enhanced selectivity[112]. The simplicity of the reaction system, with no additional reagent, contributes to the appeal of third-generation biosensors. Customization of biosensor properties is achievable through protein modification using advanced genetic or chemical engineering techniques and interfacial technologies[113]. However, challenges such as high costs and technical intricacies, including protein denaturation and renaturation in genetic engineering processes, are significant drawbacks[114]. Moreover, third-generation biosensors are still being developed and are not commonly used for analysis.
Most commercial sensors and research efforts predominantly use enzyme-based techniques from the first- and second- generations. The issue of needing an oxygen supply has been addressed using semipermeable membranes, which are both easily and cost-effectively produced. There is, however, still a strong demand for advanced sensor designs that can detect biomarkers such as glucose and lactate without requiring oxygen, offering selectivity and energy efficiency[101,115].
Immobilization of enzyme toward the fabrication of wearable biosensors
Enzyme immobilization refers to the process of constraining the mobility of an enzyme either entirely or significantly within a given space. The utilization of immobilized enzymes presents several advantages, including facile separation from the target analyte upon completion of the reaction, the ability to catalyze reactions iteratively, and the potential for multiple reuses[116]. However, biocatalysts immobilized on electrodes encounter challenges such as limited substrate accessibility and constrained mass transfer. The overall properties and efficacy of an enzyme are contingent upon factors such as the enzyme type, the immobilization matrix employed, and the methodology applied for enzyme immobilization[117]. Consequently, ensuring effective immobilization of the enzyme molecule onto the electrode is crucial for facilitating electron transport on the electrode surface. Table 3 summarizes the various immobilization methods for enzymes, highlighting their respective advantages and limitations in enhancing electrode performance. Methods for immobilizing enzymes can be either physical [Figure 2D] or chemical [Figure 2E].
Immobilization methods for enzymes
Elements | Methods | Advantages | Disadvantages | Ref. |
Physical | Adsorption | Simple process, maintains enzyme structure, Easy diffusion | Weak binding and stability, enzyme leaching, desorption due to temperature, pH, and analyte properties | [118] |
Entrapment | Simple process, minimal changes in enzyme structure, High stability, minimal enzyme demand, various matrix choices, ability to optimize the microenvironment | Enzyme leaching, mass transfer resistance, limited diffusion, relatively low enzyme loading capacity | [119] | |
Chemical | Covalent | High stability, enhanced tolerance of immobilized enzymes, minimized catalyst leakage | Enzyme structure modified, harsh conditions during immobilization, reduction of mass transfer, irreversible attachment | [120] |
Crosslinking | Good stability and durability, simple method, cost-effectiveness | Denaturing or altering the structure, delays in mass transport | [121] |
As illustrated in Figure 2D, the physical adsorption method is widely employed to develop enzyme-based biosensors due to its simplicity. Various studies have reported using this method, which involves straightforwardly applying the enzyme solution onto the electrode surface through soaking or drop-casting. The process typically includes an incubation period of overnight or 24 h to facilitate the occurrence of physical adsorption[122-125]. In this method, non-covalent linkages such as van der Waals forces, hydrophobic interactions, and hydrogen bonding allow the enzyme to adsorb onto the electrode surface without requiring pre-activation of the surface[118].
Despite maintaining the natural conformation of enzymes, this method faces notable drawbacks, including enzyme leakage and desorption triggered by changes in temperature, pH, and the nature of the analyte solution[126].
An additional example of a physical method is entrapment [Figure 2D]. In this method, enzymes are physically confined within a porous polymer matrix by linking side chains of the enzyme surface (amino acids) to the polymer surface. Importantly, the enzymes are not directly affixed to the electrode surface, allowing only the traverse of the targeted analyte and products[106]. The entrapment process involves mixing the enzyme into a monomer solution, followed by the polymerization of the monomer solution through a chemical reaction or by altering experimental conditions. Various procedures are employed in an entrapment method, depending on the entrapment type, such as electropolymerization, photopolymerization[127,128], the sol-gel process[129,130], and microencapsulation[131,132].
This method provides several advantages, including simplicity of process, preservation of intrinsic enzyme characteristics, enhanced stability, reduced enzyme leaching and denaturation, absence or required chemical modification, minimal enzyme demand, various matrix choices (e.g., chitosan, alginate, poly-acrylamide,
In addition to physical immobilization methods, chemical immobilization techniques are also explored to enhance the characterization of immobilized enzymes. Immobilizing enzymes through chemical methods involves irreversible processes where covalent or ionic bonds are established between the enzyme and the support (or surface of the electrode). One of the prevalent methods is chemical covalent bonding [Figure 2E], wherein stable functional groups on enzyme molecules interact with a support matrix[137]. The functional group on the enzyme should be non-essential for enzymatic activity, typically involving binding through side chains of the ε-amino, thiol, and carboxylic groups[137,138]. The covalent bonding process typically involves activating the support using glutaraldehyde or carbodiimide as linker molecules, followed by enzyme covalent coupling to the activated sites. Linker molecules serve as multifunctional reagents that act as bridges between the support and enzyme through covalent bonding.
This chemical bonding method has advantages, such as minimal anticipation of conformational changes when linking non-functional amino acids to the support and enhanced tolerance of immobilized enzymes to severe physical and chemical conditions (e.g., temperature, denaturants, and organic solvents). On the other hand, there are notable concerns regarding the harsh conditions during immobilization and the possibility of similar acids at the active site coinciding with the support linkage site, potentially leading to drastic changes in conformation and diminished catalytic properties of the enzyme[138].
Crosslinking stands out as another chemical method for enzyme immobilization, presenting an irreversible strategy involving establishing intermolecular crosslinks among enzymes [Figure 2E]. This process entails the creation of a robust enzyme network by forming numerous covalent bonds. Employing bi-or multifunctional reagents, such as glutaraldehyde[139,140], glyoxal[141,142], and others[143,144], achieves the desired crosslinking effect. Using cross-linkers in covalently linking enzymes to electrodes ensures heightened durability and stability, surpassing the efficacy of van der Waals or hydrophobic interactions and preventing enzyme leaching. Its widespread adoption in industrial applications is attributed to the simplicity and cost-effectiveness of the method. However, a lack of meticulous regulation in the procedure may lead to substantial enzyme loss. Furthermore, using multifunctional reagents in crosslinking introduces the risk of denaturing or altering the enzyme structure, potentially resulting in a loss of enzymatic activity. Lastly, this method contends with diffusional delays in mass transport within the system, contributing to slow reaction rate and extended equilibrium times[145,146].
Ionic binding, a less commonly employed strategy for enzyme immobilization in wearable electronics, capitalizes on ionic interactions between the charged surface of the support matrix and amino acids carrying opposite charges on the enzyme surface [Figure 2E]. The quantity of enzyme bound to the support matrix is positively correlated with the surface charge density of the materials. The support matrix materials, including polysaccharide derivatives (e.g., diethylaminoethyl cellulose[147], carboxymethyl cellulose[148], and chitosan[149]), synthetic polymers (e.g., polystyrene derivatives[150] and polyethylene vinyl alcohol[151]), and inorganic substances such as silica gel[152,153], are utilized in this method. In certain instances, physical adsorption may also occur. The key differentiation between ionic binding and physical adsorption pertains to the strength of their interactions. Ionic binding demonstrates a distinctly stronger interaction, albeit not as sturdy as that observed in covalent binding. Ionic binding offers the advantage of minimizing the conformational alterations in the enzyme structure, thereby ensuring the preservation of proper enzymatic activity. However, factors such as pH, temperature, and enzyme concentration can influence ionic interactions. Consequently, maintaining optimal ionic strength becomes imperative to prevent leaching of the immobilized enzyme in suboptimal conditions[154,155].
Non-enzymatic electrochemical sensing
Antibodies
Immunological sensors, leveraging the intrinsic specificity of antibody-antigen interactions, are instrumental in detecting biomolecules ranging from nanomolar to femtomolar concentrations[156-159]. These sensors excel in detecting large biomolecules, such as proteins and cells, by harnessing the changes in surface properties that occur upon antigen-antibody binding. Such interactions are pivotal for signal transduction, primarily through electrochemical mechanisms within the domain of electrochemical immunosensors. It is important to note that while antibodies are highly effective in detecting numerous biomolecules such as some small molecules, the specific detection of certain analytes such as glucose, lactate, potassium, sodium, and uric acid often relies on alternative sensing elements and mechanisms that may not involve traditional antibody-antigen interactions. A diverse array of methodologies is employed in this field, with the competitive and sandwich formats being particularly prominent[160]. These methodologies adeptly translate biochemical interactions into quantifiable electrical signals, thereby capitalizing on the specificity and sensitivity inherent in antigen-antibody interactions for precise biomarker detection.
The competitive format of electrochemical immunosensors [Figure 3A (i)], primarily involves label-tagged targets that compete with analytes for binding sites on specific antibodies. In this setup, the analyte and a labeled analog are introduced to the system. The more analytes in the sample, the less labeled analog binds to the antibody. The detection and quantification are negatively correlated with the analyte concentration, as the signal decreases when the analyte concentration increases. This format is particularly useful for small molecule detection where the epitope is limited, allowing for precise quantification even at low analyte concentrations.
Figure 3. Response mechanisms of non-enzymatic electrochemical sensors. (A) Scheme for aptamer-based electrochemical sensors of competitive format and sandwich format; (B) An electrochemical aptamer-based sensor blinds and releases a target molecule; (C) Scheme for the synthesis and re-binding of molecular imprinted polymers; (D) Representation of an electrochemical sensor for ion measurement having a polymeric ion-selective membrane, showing the movement of ion and charge separation that results in the surface potential which is measured.
On the other hand, the sandwich format [Figure 3A (ii)], is characterized by its utilization of two antibodies: a capture antibody and a labeled detector antibody. This method is tailored for larger molecules that possess multiple epitopes. Initially, the target analyte is captured by the immobilized antibody on the sensor surface. Subsequently, a second labeled antibody binds to a different epitope on the analyte. The sandwich format’s signal amplification, resulting from multiple bindings, enhances the sensitivity and specificity of the sensor. It is particularly effective for complex samples, providing robust detection even in the presence of interfering substances.
However, these high-precision sensors necessitate meticulous bioreagent incubation and washing steps before detection, underpinning the need for innovative solutions to streamline and adapt these processes for wearable applications[161,162].
Aptamers
Diverging from traditional antibody-based sensors, electrochemical aptamer-based (E-AB) sensors represent a significant advancement and a paradigm shift in biosensing technology. Aptamers, single-stranded DNA or RNA molecules, are discovered through the systematic evolution of ligands by exponential enrichment (SELEX) process, which involves selecting specific ligands. They are known for their ability to fold into unique spatial configurations, granting them the specificity to bind to a wide array of target molecules such as proteins, small molecules, and even cells. This binding capability is especially valuable for targets that are challenging for antibodies to recognize due to their intricate structures or other biochemical complexities[163]. This high affinity and specificity make them particularly suitable for use in sensors. A common mechanism associated with E-AB sensors is illustrated in Figure 3B. Initially, aptamers are immobilized on the electrode surface, maintaining their ability to undergo conformational changes upon binding to the target analyte. Upon introduction of the target molecule, the aptamer binds to it, undergoing a conformational change that alters the spatial orientation or the distance between the electrode surface and a reporter molecule, typically a redox-active compound, which is either part of the aptamer structure or closely associated with it. This change in spatial configuration or distance affects the electron transfer rate between the redox reporter and the electrode, a phenomenon meticulously captured by the sensor. Specifically, the target binding event leads to either an increase or decrease in the electron transfer rate, depending on the nature of the conformational change. This, in turn, causes a change in the electrochemical signal, typically measured as a change in current, potential, or impedance. The electrochemical signal is then recorded and quantified, correlating directly with the concentration of the target analyte in the sample. The specificity of the aptamer for its target ensures that the sensor response is highly selective, while the versatility of the aptamer structure allows for the detection of a wide range of targets, from metal ions[164,165] to protein molecules[166,167]. Despite the impressive capabilities of E-AB sensors, challenges such as the necessity for specific aptamer sequences for each target and potential for non-specific binding do exist[168]. However, ongoing advancements in aptamer selection[169-171], strategies for preventing non-specific binding[172], and immobilization strategies[173,174] are progressively refining these sensors, enhancing their specificity, sensitivity, and practical applicability.
MIPs
MIPs are engineered as synthetic receptors, offering a high specificity for target molecules in electrochemical biosensing applications. The synthesis of MIPs entails arranging monomers around a preselected template molecule. Following polymerization and the subsequent removal of the template, the process yields tailored cavities that correspond in shape and functional group orientation to the target molecule. These cavities serve as binding sites for the analyte, causing a detectable electrochemical signal shift that is directly proportional to the analyte concentration in the sample[175].
Figure 3C delineates the MIP synthesis stages. The process begins with the strategic assembly of functional monomers around a template molecule. After polymerization, with a cross-linker to reinforce the structure, the template is extracted, leaving behind cavities that are primed for the selective re-association with the target analyte[176].
In the context of electrochemical biosensing, MIPs offer several advantages over biological receptors such as antibodies and aptamers. Unlike these biological counterparts, MIPs are synthesized to be inherently more stable across a broad spectrum of environmental conditions, including extreme pH, temperatures, and solvent compositions[177]. Their robust nature ensures longevity and reusability, which are significant benefits for practical applications.
However, MIPs also exhibit certain limitations. Their inherent insulating nature can inhibit sensitivity due to poor mass transfer, or challenges such as incomplete removal or saturation of the MIP matrix when exposed to a sample containing the target[178]. These issues not only affect the sensitivity of the sensor but also its reusability, often necessitating design modifications to enhance performance.
Antibodies, Aptamers, and MIPs as bioaffinity elements, along with their target specificity, stability, and cost-effectiveness in various sensing applications, are comparatively analyzed in Table 4. This detailed comparison aims to equip researchers with a clearer insight into selecting the most suitable bioaffinity element for their specific sensor applications.
Comparative analysis of MIPs, aptamers, and antibodies as bioaffinity elements
Elements | Antibodies | Aptamers | MIPs |
Analyte | Small molecules Proteins and cells Peptides | Small molecules Proteins | Small molecules |
Target specificity | Very high | High | High |
Stability | Low (sensitive to temperature and pH changes) | High (variable) | High |
Production cost | High | Moderate | Low |
Advantages | High specificity and selectivity, broad target recognition, commercial availability | Chemically stable, facile modification, high sensitivity and reproducibility | Highly specificity and reproducibility, environmental durability, low cost |
Disadvantages | Low stability, limited reusability, difficulty in modification, protein denaturation, high cost | Non-specific binding, restricted selection of sequences with good sensitivity | Relatively low binding performance, cross-selectivity, leakage of template, challenges with large targets (proteins and cells) |
Ref. | [161] | [179] | [180] |
Ion-selective membrane
Ion-selective electrodes (ISEs) constitute a well-established potentiometric sensor technology extensively applied across environmental, industrial, and clinical domains to analyze crucial electrolytes[181-183]. The burgeoning field of wearable sensors is currently centered on using ISEs for real-time, non-invasive monitoring of ions in biological fluids, showing recent advancements geared toward evaluating personal physiological states. Potentiometric sensors, specifically designed for ion sensing, have garnered attention owing to their compact size, exceptional selectivity, prompt response, low energy consumption, user-friendly operation, and economic viability[184,185].
These potentiometric sensors, comprising a WE and RE, are presumed to have their potentiometric response modeled under open-circuit state, specifically under the condition of zero current. In ISEs, the analytical insight is derived by translating an ion-exchange event into a voltage signal[100]. The electrode design focuses on perturbations in the local equilibrium at the interface between an ISM and the sample solution. Variations in the activity of the primary ion induce changes in membrane potential and that provided by the RE[185,186] [Figure 3D].
The potential response of ISEs can be explained by the phase-boundary potential mode, which is based on total equilibrium assumptions[187,188]. It relies on charge separation at the solution-membrane interface, influenced by primary ion activity through surface chemisorption and equilibrium partitioning of primary ions. The energy required for charge separation stems from the chemical driving force, determined by Gibbs free energy. Through the perselectivity and ion selectivity of ISMs, primary ions can move across the membrane phase unhindered. Upon reaching a state where chemical driving forces counterbalance the opposing electrical Coulombinc driving forces, electrochemical equilibrium is attained, forming an electrical double layer and establishing a potential difference. The basic functional relationship between the potential and activity follows the Nernst equation, denoted as[188-190]
where zi is charge on the ion, Q is activity in the solution, E0 is the standard potential, R is the universal ideal gas constant (8.134 J·K-1·mol-1), T is temperature in Kelvins, and F is the Faraday constant
ISMs encompass several crucial constituents, including a matrix/supporting material, plasticizer, anion/cation excluder, and the ionophore. The matrix/supporting material, often high molecular weight polyvinyl chloride (PVC), is chosen for its low toxicity, chemical inertness, and strength. The plasticizer is pivotal in facilitating the integration of the ionophore into the polymeric matrix, thereby exerting significant influence over the ion adsorption/absorption dynamics and the resultant redox characteristics. These characteristics may manifest either in a Nernstian response, characterized by equilibrium partitioning of analyte ions between the sample and the membrane at their interface, or in a non-Nernstian manner, typified by a nonequilibrium steady state condition[191-193]. The dielectric constant of the plasticizer is crucial, and the redox behavior of ISMs is highly sensitive to plasticizer chemistry and concentrations. Anion/cation excluders are employed to minimize competitive coordination between the ionophore and the counter ion of the analyte. The sensing component, the ionophore, is responsible for ion immobilization, and the redox/double layer capacitance at the ISM-electrode substrate interface, which is determined by the inherent characteristics of WE composed of functional materials acting as ion-to-electron transducers, is expected to demonstrate a stable potentiometric response to changes in ion activity[194,195].
APPLICATIONS OF WEARABLE ELECTROCHEMICAL SENSOR FOR DIABETES
DM is a global health concern linked to metabolic syndrome, necessitating continuous monitoring of blood glucose levels for effective diagnosis and management[196,197]. The urgency arises from potential complications such as cardiovascular disease, stroke, kidney disease, and mortality associated with DM[9-14,198-201]. Regularly assessing biomarkers such as glucose, lactate, ketone, insulin, and electrolytes (Na+ and K+) is crucial for a proactive approach to prevent and manage DM and its complications. Non-invasive wearable electrochemical sensors are key in quantifying these biomarkers in easily accessible bodily fluids such as sweat, tears, saliva, and ISF[97]. Given the importance of selecting the most suitable biological matrix for continuous monitoring in DM management, we present a comparative analysis to guide the development and application of wearable electrochemical sensors. The following table summarizes our assessment of various biological matrices, highlighting their advantages, disadvantages, suitability for diabetes management, and relevant references [Table 5]. The non-invasive wearable electrochemical sensors typically employ a three-electrode system for redox reactions, with a nanotextured WE surface to enhance signal-to-noise ratio (SNR) and lower detection limits[89-91,208]. Selectivity is augmented by coating the nanotextured surface with specific functional groups such as enzymes, antibodies, aptamers, and designed peptides, along with selecting appropriate voltage potentials for reaction activation[51,209]. Wearable sensing devices, designed for real-time monitoring of various biomarkers, offer valuable insights into the health status and fitness levels of DM patients. This summary highlights advancements in designing wearable electrochemical biosensors for monitoring DM-associated biomarkers in bodily fluids.
Advantages and disadvantages of various matrices in diabetes management
Matrix | Advantages | Disadvantages | Suitability for DM | Ref. |
Sweat | Non-invasive, potential for continuous monitoring | Low concentration, contaminants through skin, periodic activation of sweat glands | Moderate correlation with blood glucose | [202,203] |
Tears | Non-invasive, minimal discomfort | Low concentration, hard implementation, potential corneal injury | Further study of the correlation is necessary | [204,205] |
Saliva | Non-invasive, easy to collect | Low concentrations, large amounts of impurities | Moderate correlation with blood glucose | [101,206] |
ISF | Minimally invasive, good correlation with glucose levels | Slight delay in glucose level changes compared to blood | High correlation with blood glucose | [207] |
Sweat
Sweat plays a crucial role in regulating the body temperature and offers valuable insights into human health and physiological conditions by continuously excreting non-invasive and biologically relevant biomarkers. It is a favorable biofluid for non-invasive biosensing of analytes due to its abundant composition of detectable biomolecules and electrolytes, providing valuable information for DM management.
Pirovano et al. introduced SwEatch, a 3D-printed wearable sensor for real-time electrolyte monitoring in sweat, emphasizing Na+ and K+ concentrations[210]. The band-type platform of SwEatch incorporates a dual macro-duct for direct monitoring, comprising three replaceable components: microfluidic unit, platform body, and fully integrated wearable platform. The microfluidic unit features a dual macro duct for independent sample channels [Figure 4A]. Electrode fabrication involves screen printing layers on Polyethylene terephthalate (PET) sheets, using solid contact ISEs with poly(3,4-ethylenedioxythiophene) (PEDOT) or poly(3-octylthiophene-2,5-diyl) (POT) as conductive polymers. POT streamlines fabrication, enhancing reproducibility through partial automation. These electrodes exhibit sensitivity and selectivity towards sweat interferents (H+, Na+, K+, Mg2+, Ca2+). The 3D printed SwEatch, with a mirrored fluidic unit, utilizes passive capillary action to bring sweat to two independent electrodes. On-body trials during cycling reveal sodium (1.89-2.97 mM) and potassium (3.31-7.25 mM) concentration increases over a 90-minute exercise period [Figure 4A]. Potentiometric signals are measured, digitized, and transmitted via Bluetooth, showcasing the potential of SwEatch for real-time electrolyte monitoring during physical activity.
Figure 4. Sweat-based wearable electrochemical sensors for diabetes mellitus. (A) Scheme of band-type ion sensor for Na+ and K+ from sweat, incorporating microfluidic unit, platform body, and the fully integrated wearable platform. The image showing the positioning SwEatch platform on the arm during the on-body trials and the corresponding outputs measuring Na+ and K+. Reproduced with permission[210]. Copyright 2020, Elsevier B.V.; (B) Optical image of the headband-type wearable biosensor for on-body sweat sensing during physical activities and its corresponding measurements of Na+ and Lactate. Reproduced with permission[211]. Copyright 2021 Elsevier B.V.; (C) Photographs and schematic illustration of a sweatband fluidic platform and real-time data obtained from on-body Na+ monitoring during indoor cycling. Reproduced with permission[212]. Copyright 2017, American Chemical Society; (D) Photographs of biofuel cell array integrated on stretchable textile and on-body test during the cycling exercise with its corresponding potentiometric response to the lactate. Reproduced with permission[213]. Copyright 2016, The Royal Society of Chemistry; (E) Optical and schematic images of the biosensing patch on the forehead. The corresponding real-time measurements of glucose from sweat and sweat rate from one healthy and one diabetic subject. Reproduced with permission[214]. Copyright 2019, American Association for the Advancement of Science.
Zhao et al. introduced a wearable headband nanobiosensor integrating conductive threads embedded with zinc-oxide nanowires (NWs), designed for on-body detection of sweat lactate and Na+ during physical exercise [Figure 4B]. The biosensor incorporates signal readout and data communication circuits, enabling precise and wireless monitoring of human sweat. With detection ranges covering clinically relevant concentrations (0-25 mM for lactate and 0.1-100 mM for sodium) and limits of detection at 3.61 and
Jeerapan et al. reported a study on highly stretchable textile-based biofuel cells, employing customized stress-resistant inks through screen-printing[213]. These bioelectronic devices, resembling socks in their design, were crafted with nanomaterial-based inks and incorporated serpentine patterns, showcasing notable resilience to substantial mechanical deformations, including stretching and twisting [Figure 4D]. In the glucose and lactate biofuel cells, employing a setup featuring a sole enzyme and absence of a membrane, remarkable maximum power densities of 160 and 250 μW·cm-2 were observed, alongside open-circuit potential of 0.44 and 0.46 V, respectively. The textile-based biofuel cells maintained their structural integrity even after enduring repeated severe mechanical deformations, illustrating a consistent and stable power output through 100 cycles of 100% stretching. This self-powered biosensing system exhibited minimal background signals and sustained operational stability for 50 min under physiological conditions, highlighting its potential for the self-driving logic development in Biocomputing systems and simplified design of mechanically compliant intelligent wearable electronics.
Wearable sweat sensors have shown promise for on-site measurements, offering potential for preventive healthcare and prompt diagnosis. However, comprehensive studies are crucial to establish diagnostic effectiveness and constraints. Overcoming the challenge of extensive population studies requires high-throughput fabrication of uniform, dependable sweat sensors with minimal preparatory requirements, enabling simultaneous regional measurements in multisubject and longitudinal trials.
Nyein et al. introduced a high-throughput wearable microfluidic patch for sweat analysis during exercise and iontophoretic sweat conditions[214]. The patch, using advanced printing and cutting techniques, allows uniform production and reveals correlations between sweat secretion rate, sodium levels, and hydration status. In iontophoretic sweat, individual-specific correlations between sodium and potassium levels are identified. The study explores the connection between iontophoretic sweat glucose and blood glucose for healthy and diabetic subjects, emphasizing personalized correlations over universal thresholds for diabetes diagnostics [Figure 4E]. Continuous monitoring of glucose dynamics in the iontophoretic sweat of both healthy individuals and those with diabetes reveals varied patterns. In trials involving healthy subjects, the sweat glucose levels decline from 80 to 72 μM in the initial trial, corresponding to a drop in blood glucose from 85 to 79 mg/dL. However, in the subsequent trial, despite a higher average sweat glucose level, it increases from 112 to 122 μM, while blood glucose decreases by 16 mg/dL. Notably, the decrease in sweat rate occurs earlier in the first trial. Conversely, in the case of the diabetic subject, the sweat rate remains consistent, and the sweat glucose levels mirror the trend observed in blood glucose, rising in the first trial and declining in the second.
The research findings suggest that the lack of a consistent association between sweat and blood glucose levels among different individuals presents a hurdle in establishing standardized sweat thresholds for the diabetes diagnosis or treatment. Unraveling the potential for personalized connections between sweat and blood glucose may require extensive longitudinal investigations into the dynamics of sweat glucose. Additionally, future studies should delve into sweat glucose variation patterns among subjects, intra-individual regional disparities, and the influence of exercise and iontophoresis-induced sweat rate and stimulation duration on glucose levels in sweat.
Tear
Tears offer a promising avenue for non-invasive glucose monitoring due to their easy accessibility, continuous flow, and correlation with blood glucose levels. This has increased interest in developing wearable tear glucose sensors that utilize optical and electrochemical detection methods[215-219]. Electrochemical detection, in particular, stands out for its rapid response, high sensitivity, and accuracy. Additionally, considering the delicate nature of the eye, these wearable tear-based sensors must prioritize safety and user comfort by being nontoxic, flexible, and miniaturized. Based on these requirements, several trials were conducted to develop electrochemical biosensors based on tear analysis. As shown in Figure 5A, a microneedle-based amperometric electrochemical glucose sensor was developed to collect microliter volumes of tears with capillary tubes[220]. The sensor consisted of platinum/iridium (Pt/Ir) wire immobilized with GOX and inner layers of Nafion and an electropolymerized film of 1,3-diaminobenzene/resorcinol for enhancing the selectivity of glucose. The developed glucose sensor demonstrated a sensitivity of
Figure 5. Tear-based wearable electrochemical sensors for diabetes mellitus. (A) Scheme of needle-type tear glucose sensor. Reproduced with permission[220]. Copyright 2011, American Chemical Society; (B) Design of flexible glucose sensor attachable to contact lens. Reproduced with permission[221]. Copyright 2010, Elsevier B.V.; (C) Optical image of a contact lens integrated with amperometric glucose sensor. Reproduced with permission[222]. Published by Elsevier B.V.; (D) Integrated transparent contact lens capable of transmitting data wirelessly and measuring glucose and Intraocular pressure. Reproduced with permission[223]. Copyright 2017, The Authors; (E) Schematic representation of a soft, wireless contact lens with integrations of electrochemical glucose sensor and display that serve as feedback indicators. Reproduced with permission[224]. Copyright 2017, The Authors; (F) Smart contact lenses for continuous glucose monitoring and treatment of diabetic retinopathy. Reproduced with permission[225]. Copyright 2017, The Authors; (G) A wearable tear-collecting eyeglasses system with a microfluidic electrochemical detector. Reproduced with permission[226]. Copyright 2019 Elsevier B.V. PDMS: Polydimethylsiloxane; f-DDS: flexible drug deliver system; CE: counter electrode; WE: working electrode; RE: reference electrode.
Saliva
Saliva is a key and easily accessible biofluid for non-invasive health monitoring, containing diverse biomarkers such as glucose, lactate, hormones, and electrolytes[227,228]. It primarily originates from salivary glands, reflecting contributions from various sources. Establishing correlations between blood and saliva analyte concentrations is a common practice[229,230]. Recent research focuses on developing wearable electrochemical biosensors for real-time saliva monitoring, particularly in glucose analysis for diabetes diagnostics. Salivary glucose levels correlate with plasma levels, suggesting a potential painless and non-intrusive approach to diabetes monitoring[231]. However, challenges include the need for highly sensitive sensors due to lower glucose concentrations in saliva, ensuring full biocompatibility for oral placement, and addressing potential interference from elevated protein content or active compounds of saliva from food residues. These multifaceted challenges hinder the seamless development of robust and reliable wearable salivary glucose sensing devices.
In the pursuit of creating a durable, dependable, and biocompatible electrochemical sensor wearable for monitoring saliva glucose levels to aid in diabetes management, Bihar et al. have reported the development of an enzymatic glucose sensor[232]. Notably, this sensor is inkjet-printed on paper substrates, showcasing a novel approach in the ongoing efforts to advance diabetes-related biosensing technologies. The device fabrication involved utilizing a commercially available PEDOT:polystyrene sulfonate (PSS) ink (conductivity of 250 S/cm) that is suitable for inkjet printing. The configuration employed a three-electrode system on glossy commercial paper [Figure 6A]. The biorecognition element, consisting of GOX and a Fc complex, was incorporated by printing an aqueous solution onto the WE. Fc, serving as an electron mediator, enhances sensor selectivity and widens the operational range by molecularly connecting the enzyme to the sensing electrode. However, due to the weak adhesion on surfaces and its potential toxicity concerns, Fc is mixed with the polysaccharide and chitosan in a solution. A thin layer of Nafion was applied to the three electrodes (WE, RE, and CE) as a robust barrier against potential interference from complex biological environments or unspecified redox reactions during electrode operation. The device exhibits operational capability within a range spanning from 0.025 to 0.9 mM, showcasing efficient sensitivity towards glucose concentrations present in saliva. This sensitivity makes it suitable for detecting abnormal glucose levels during screening processes. Even after one month of storage at room temperature under vacuum, the sensors retain functionality, experiencing minimal performance loss (< 25%).
Figure 6. Saliva-based wearable electrochemical sensors for diabetes mellitus. (A) Optical image of the inkjet-printed glucose biosensors coated with the enzyme and the mediator (GOX and Fc) and encapsulated with Nafion. Reproduced with permission[232]. Copyright 2018, The Author(s); (B) Optical images of the screen-printed WE, RE and CE for electrochemical sensor on the mouthguard. Reproduced with permission[233]. Copyright 2018, Elsevier B.V.; (C) Optical images showing the integration of the wireless mouthguard glucose sensor and its real-time response when installed in the human oral cavity. Reproduced with permission[234]. Copyright 2020, American Chemical Society; (D) The overview of a non-invasive, wireless pacifier, inserted into a baby model and its Na+-to-K+ ion ratio. Reproduced with permission[235]. Copyright 2022, Elsevier B.V.; (E) The overview images of glucose pacifier-biosensor sensing concept. Signal interpretation: a dry device (I), saliva reaches and starts to fill the electrochemical chamber (II), stabilization of the signal (III), glucose signal (IV), and saliva elimination from the pacifier (V). Reproduced with permission[236]. Copyright 2019, American Chemical Society. GOX: Glucose oxidase; Fc: ferrocene; WE: working electrode; RE: reference electrode; CE: counter electrode.
In pursuing diabetes management and prevention, a prevalent avenue involves developing wearable devices, particularly in the form of mouthguards. These devices serve as user-friendly tools for real-time monitoring of diabetes-related substances found in saliva. Ciui et al. introduce a cavitas-printed electrochemical sensor for directly detecting salivary components[233]. The sensor, characterized by high flexibility and bendability, is seamlessly integrated into a customized mouthguard positioned on a simulated jaw structure resembling the human oral cavity. The disposable nature of the sensor allows easy attachment to and detachment from the mouthguard, facilitating replacement as needed. The use of cost-effective printing techniques, rapid measurement times, prolonged storage stability, and user-friendly operation adds to the attractiveness of this innovative mouthguard sensor [Figure 6B]. Moreover, as depicted in Figure 6C, Arakawa et al. reported a glucose sensor incorporated into a mouthguard design, enabling wireless monitoring of salivary glucose concentration through a mobile terminal[234]. The mouthguard sensor, evaluated using artificial saliva, demonstrates the capability to measure glucose concentrations within the range of 1.75-10,000 μmol/L, covering salivary sugar concentrations from 20 to 200 μmol/L. Also, applying a cellulose acetate membrane on the electrode functioned as an interference rejection membrane, effectively mitigating the influence of contaminants such as ascorbic acid and uric acid, achieving a notable noise ratio suppression of 97.1%. In
Explorations into health monitoring through saliva are broadening to encompass infants. There is ongoing research in creating wireless pacifiers for the early monitoring of childhood health and for managing and preventing diabetes-related conditions. Lim et al. presented a bioelectronic pacifier with smart, wireless capabilities for monitoring salivary electrolytes in neonates[235]. This device enables real-time, continuous detection of Na+ and K+ levels [Figure 6D]. Incorporating ion-selective sensors, flexible circuits, and microfluidic channels, the portable device enables simplified procedures for non-invasive electrolyte monitoring. The flexible microfluidic channel allows for continuous and efficient collection of saliva from the oral cavity. Garcia-Carmona et al. have introduced a fully integrated wearable pacifier-based platform designed for wireless non-invasive monitoring of glucose in the saliva of newborns[236]. The biosensor utilizes enzymatic detection based on GOx on a Prussian Blue electrode transducer. The pacifier sensing platform combines wireless amperometric circuitry with a Bluetooth communication system to achieve compactness and low-power operation [Figure 6E]. Featuring an isolated electrochemical detector to prevent material leakage into the mouth, the device also includes a polymeric nipple equipped with a safety rectifying channel for saliva sampling. As presented in Figure 6E, saliva measurements from diabetic patients were conducted using the pacifier sensor 30 min post-meal. The signal observed in a fasting state (b) was lower than after a meal (c). Furthermore, the ability of the device to detect glucose levels in diabetic adults was examined and compared to their blood glucose levels, revealing a strong correlation and affirming the effectiveness of the sensor.
ISF
In recent decades, a pronounced focus has been on developing wearable electrochemical sensors to monitor ISF beneath the epidermal layer. This fluid, rich in essential ions, such as Na+, K+, and Cl-, and metabolites, including glucose and lactate, has emerged as a pivotal target for obtaining precise and rapid data on glucose levels, rivaling blood as a primary source. ISF encapsulates the skin cells, facilitating nutrient supply through direct diffusion from the capillary endothelium[51]. This physiological mechanism establishes reliable associations between the levels of various biomolecules in the blood and ISF, except for larger molecules such as lipids[237]. Given its ease of accessibility and robust correlation with the established gold standard of blood sampling, a range of miniaturized wearable devices is designed for real-time sensing of ISF[237-240]. Notably, commercially available options include Abbott’s FreeStyle Libre, DexCom G6, GuardianTM Sensor 3, A.Menarini Diagnostics GlucoMen Day CGM, and ISENS Caresens Air, specifically designed for monitoring glucose levels in individuals managing diabetes [Table 6].
Commercially available wearable electrochemical glucose sensor for DM management
Biofluids | Company | Enzyme | Lifetime (days) | Detection range | Calibration (fingersticks) | Warm-up (minutes) |
ISF | Abbott FreeStyleLibre3 | GOX | 14 | 40-500 mg/dL | - | 60 |
ISF | DexCom G7 | GOX | 10 | 40-400 mg/dL | - | 30 |
ISF | Medtronic GuardianTM Sensor 3 | GOX | 7 | 50-400 mg/dL | - | 40 (up to 120) |
ISF | A.Menarini Diagnostics GlucoMen Day CGM | GOX | 14 | 40-400 mg/dL | 1/day | 55 |
ISF | ISENS Caresens Air | GDH-FAD | 15 | 40-500 mg/dL | 1/day | 120 |
MNAs offer a minimally invasive approach for monitoring vital biomarkers in ISF. Sharma et al. developed a glucose biosensor with a MNA electrode for continuous monitoring. The sensor devices were crafted through casting structures in SU8 50, subsequent crosslinking, and metallization using platinum or silver for the WE and RE, respectively. Functionalization included entrapping GOX within an electropolymerized polyphenol (PP) film. Scanning electron microscopy (SEM) images revealed MNAs with dimensions of 1,000 μm length, 600 μm base, 35 μm tip diameter, and 1,200 μm pitch. In Figure 7A, the sensor exhibited a surge in current, reaching 4,500 nA in response to a glucose bolus (slice of cake) over 45 min, followed by a decline. The figures demonstrated a correlation and time lag between capillary blood and dermal ISF. Despite the focus on a minimally invasive design, in-vivo studies revealed operational challenges in securely fixing the sensors in the sub-dermal space. Figure 7A on the right visually depicted artifacts from arm movement, with a shorter duration than observed changes in glucose concentration[241].
Figure 7. ISF-based wearable electrochemical sensors for diabetes mellitus. (A) Optical and SEM images of microneedle array electrodes and in-vivo data obtained after positioning the microneedle array electrodes on the forearm. Reproduced with permission[241]. Copyright 2016, The Author(s); (B) The optical and illustration images of wireless CGM system paired with smartphone app. The associated data comprises continuous monitoring of ISF glucose levels over an extended period using the wireless CGM system, compared against glucose readings obtained via a glucometer on days 1, 7, and 14. Reproduced with permission[242]. Copyright 2023, American Chemical Society; (C) The optical image of non-invasive blood glucose sensors attached on the wrist, and its on-body blood glucose sensing properties of the non-invasive glucose sensors attached on hand back of healthy volunteers. Reproduced with permission[244]. Copyright 2021, Elsevier B.V.; (D) Photographs of wearable patch for glucose monitoring in ISF on the skin. The data compares hourly normalized ISF glucose (blue bars) and blood glucose (red bars) profiles. The line plot depicts the real offset between ISF glucose (blue line) and blood glucose (red line) obtained from the differences in monitoring each biofluid. Reproduced with permission[245]. Copyright 2021, American Chemical Society. CGM: Continuous glucose monitoring; ISF: interstitial fluid; SEM: scanning electron microscopy.
Yang et al. developed a smartphone-controlled wearable continuous glucose monitoring (CGM) system using microneedles[242]. The system featuring a disposable glucose sensor and a reusable CGM device employs a cost-effective fabrication method involving screen printing and laser cutting for double-sided electrochemical three-electrode strips. The enzyme immobilization strategy uses a sandwich-type configuration, including a protective biocompatible membrane and layers with carbon nanotubes (CNTs) and Nafion. Glucose level fluctuations were monitored on day 1, day 7, and day 14 [Figure 7B], revealing a rapid post-injection increase and return to normal levels within one hour in healthy rats. The CGM system demonstrated similar profiles to a standard glucometer, with ISF glucose levels showing a typical delay of approximately 10 min, attributed to dermal capillary density and slow epidermal metabolic activity.
Reverse iontophoresis (RI), recognized as another method for monitoring ISF nutrients, is an advanced non-invasive technique involving extracting ISF to the skin surface using electrical currents through skin-worn electrodes. This process induces an ion flux, primarily cations such as Na+, across the skin, creating an electroosmotic flow that transports neutral glucose molecules toward the cathode electrode[243].
Yao et al. presented a non-invasive blood glucose sensor featuring a flexible and wearable design [Figure 7C]. Utilizing a graphene (G)/CNTs/GOx composites textile as the WE and a G/CNTs/Ag/AgCl as the CE[244], the device was evaluated for its effectiveness in non-invasively measuring glucose levels in humans. This assessment involved placing the sensors on the wrists of three healthy volunteers [Figure 7C]. The response current densities of the wrist-mounted sensors indicated that the calculated blood glucose concentrations closely matched those measured by a standard glucose meter for all three volunteers. Notably, the two-electrode non-invasive glucose sensors exhibited the capability for intermittent glucose monitoring over an extended period of more than 8 h.
De la Paz et al. reported a flexible platform for non-invasive ISF extraction and detection, resembling a temporary tattoo, with an extended sensing operation time of 8 h[245]. The platform integrates with an electronic board for controlling the RI-sensing operation and enables real-time wireless transmission, making it suitable for extended daily glucose monitoring. This was demonstrated in a clinical investigation involving individuals with diabetes [Figure 7D]. The wearable glucose patch was tested for 4 h in diabetic patients, with blood and ISF glucose recordings following protocols used in initial eight-hour tests. During fasting, all blood glucose measurements exceeded 100 mg/dL [Figure 7D], indicating higher ISF glucose levels than healthy volunteers. The experiments showed minimal changes in glucose levels during the first two measurements (0:20 and 1:00 h), followed by a significant rise 1 h after food ingestion (2:00 h). The consistent agreement between ISF and blood readings confirms the reliability of non-invasive glucose measurements for extended monitoring.
MULTIPLEXED WEARABLE SENSOR FOR DIABETES MELLITUS
Multiplexed analysis of wearable sensor
In recent years, significant strides have been taken in developing multiplexed wearable sensors for DM monitoring. These advanced platforms enable the simultaneous analysis of various diabetes biomarkers, providing a comprehensive picture of the individual health status. One direction of multiplexed analysis involves the integration of electrochemical and physiological biomarkers including cardiovascular and/or physical parameters. This multiplexed approach offers real-time insights into not only DM management but also complications resulting from it. Another promising avenue of multiplexed analysis centers on simultaneously measuring multiple electrochemical biomarkers. These sensors enable the concurrent monitoring of glucose and other vital biomarkers in single- or multi-human biofluid. Such simultaneous monitoring is necessary to understand the potential synergistic effects and complicated fluctuation of DM-related biomarkers.
Electrochemical and physiological biomarkers
As the pioneering study for multiplexed analysis of electrochemical and physiological biomarkers, the hybrid wearable patch was developed to simultaneously measure biochemical and electrophysiological parameters [Figure 8A]. Patch-type electrodes for the lactate biosensor and electrocardiogram were fabricated by leveraging a screen-printing method on the polyester sheet. Human experiments collecting the hybrid signals during 15-30 min cycling activity demonstrated concurrent lactate and heart rate measurement, extracted from electrocardiogram data, with negligible cross-talk[246]. In addition to patch-type wearable sensors, an integrated wearable health management system with a glucose sensing strip and smart band was reported in 2018 [Figure 8B]. A sweat-based glucose sensing strip was attached on the forehead, and a smart band with an electronic circuit was worn on the wrist for measuring physiological signals, such as blood oxygen saturation level, heart rate, and physical activity. The sensing strip, which collected the sweat, was inserted into the smart band for electrochemical glucose measurements. Daily activity studies with human subjects showed pre- and post-exercise monitoring of glucose and physical activities, which is important to prevent hypoglycemic shock during a high-intensity workout[247]. Additionally, an ear-worn device was presented as one of multiplexed wearable platforms [Figure 8C]. Sweat-based lactate concentration and pH levels were measured using amperiometric and potentiometric sensors around both ears. At the same time, electrocardiogram (ECG) was recorded around the ears with a SNR level of 18 dB. Acquired signals were transmitted wirelessly to the mobile phone via Bluetooth Low Energy (BLE) communication[248]. These wearable sensing platforms have limitations in reducing their sizes due to batteries for power supply. As shown in Figure 8D, Yu et al., from the group led by Gao, reported the fully perspiration-powered integrated electronic skin (PPES) operated by highly efficient lactate biofuel cells with a power density of 3.5 mW/cm2. During a 60-hour operation, the PPES monitored multiplexed signals including metabolic analytes and the skin temperature. Moreover, with a CNTs-PDMS elastomer-based strain sensor, on-body evaluation of the PPES was performed by measuring muscle contraction and validated the promising use of human-machine interaction for the prosthetic control[249]. In addition to electrical sensing methods for measuring physical signals, acoustic sensing with ultrasonic transducers was used to acquire more complex hemodynamic parameters. As shown in Figure 8E, a wearable device could continuously and simultaneously monitor multiple biomarkers via enzymatic chemical sensors and physical parameters, such as blood pressure and heart rate, via ultrasonic transducers. Glucose in ISF and lactate, caffeine and alcohol in sweat were measured during the different daily activities. This multiple analysis demonstrated that these skin-worn devices could ensure the understanding of various activities, such as food or caffeine intake, digestion and exercise, and the forecasting of physiological changes[250].
Figure 8. Multiplexed biosensors coupling electrochemical and physiological biomarkers for monitoring diabetes mellitus. (A) A chemical-electrophysiological hybrid bio-sensing system with screen-printed lactate sensor and ECG electrodes. Reproduced with permission[246]. Copyright 2016, The Authors; (B) A wearable smart band for detecting sweat-based glucose and vital signs. Reproduced with permission[247]. Copyright 2016, Springer Nature Limited; (C) A wireless ear-worn device capable of pH, lactate and ECG sensing during physical exercise. Reproduced with permission[248]. Copyright 2018, WILEY-VCH Verlag GmbH & Co. KGaA, Weinheim; (D) Scheme of biofuel-powered soft biosensor with multiplexed metabolic sensing system. Reproduced with permission[249]. Copyright 2020, The American Association for the Advancement of Science; (E) An epidermal patch capable of monitoring sweat-based lactate, caffeine, alcohol and ISF-based glucose and ultrasound-based hemodynamic information. Reproduced with permission[250]. Copyright 2021, The Authors, under exclusive license to Springer Nature Limited, part of Springer Nature. WE: Working electrode; RE: reference electrode; CE: counter electrode; ISF: interstitial fluid; ECG: electrocardiogram.
Multi-electrochemical biomarkers
For more accurate analysis using a wearable non-invasive biosensor, Gao et al., from the group led by Javey, showed the fully integrated biosensor array for in situ perspiration measurement, which is integrated with functional components such as conditioning, processing and wireless transmission in the wristband platform and measures sweat metabolites of glucose and lactate and electrolytes of potassium and sodium [Figure 9A]. On-body perspiration analysis during cycling activity demonstrated real-time and selective measurement of multi-electrolytes and metabolites of a human subject wearing a smart headband and a smart wristband. In addition to flexible band-type configurations, various efforts have been made to develop a form of wearable biosensor that can be easily integrated with existing accessories[34]. As shown in Figure 9B, Sempionatto et al., from the research group led by Wang, presented a fully integrated eyeglasses platform for real-time monitoring of glucose, lactate and potassium in sweat. The screen-printed electrochemical sensors were placed on the nose pads of eyeglasses, and a wireless electronic system was attached on the arms of the eyeglasses frame[251]. Efforts toward in-vivo sensing of multiplexed electrochemical parameters involved developing needle-based microscale biosensor arrays [Figure 9C]. Active layers were electrodeposited on a polymeric polyimide substrate and integrated into the curved surface of the medical needle. By measuring real-time glucose, lactate concentration, electrical conductivity and pH, the needle-based biosensor array discriminated cancer from the normal tissues[252]. For improving the measurement accuracy of multiplexed glucose and lactate sensing, Yokus et al., from the group led by Daniele, designed the wristwatch-shaped analog front-end of the electrochemical cells capable of measuring twelve WE [Figure 9D]. Each WE collected chronoamperometric responses, and an accurate measurement was obtained as an average of all collected data. This measurement strategy showed sensitivities of
Figure 9. Multiplexed biosensors coupling multi-chemical biomarkers for monitoring diabetes mellitus. (A) Fully integrated sensor arrays for multiplexed monitoring of glucose, lactates, sodium, potassium and skin temperature in sweat. Reproduced with permission[34]. Copyright 2016, Springer Nature Limited; (B) Eyeglasses-based sensor platform for monitoring glucose, lactate and potassium ions. Reproduced with permission[251]. Copyright 2017, Royal Society of Chemistry; (C) A flexible biosensor integrated with curved needle for the measurement of electrical conductivity, pH, glucose and lactate. Reproduced with permission[252]. Copyright 2020, American Chemical Society; (D) A band-type wearable platform with flexible sensor array for simultaneous monitoring of glucose, lactate, pH, and temperature. Reproduced with permission[253]. Copyright 2020, Elsevier B.V.; (E) Schematic representation of microneedle platform for real-time continuous glucose/ketone monitoring in ISF. Reproduced with permission[257]. Copyright 2020, American Chemical Society; (F) Touch-based β-HB/glucose sweat sensing device for personalized health monitoring. Reproduced with permission[258]. Copyright 2022, American Chemical Society; (G) A microfluidic chip integrating dual assays for simultaneous measurements of glucose and insulin. Reproduced with permission[259]. Copyright 2019 Wiley-VCH Verlag GmbH & Co. KGaA, Weinheim; (H) Portable aptamer-based biosensor chip for non-invasive simultaneous monitoring of glucose and insulin. Reproduced with permission[260]. Copyright 2022, Elsevier B.V. All rights reserved; (I) Epidermal patch-type biosensor platform capable of simultaneous alcohol sensing in sweat and glucose sensing in ISF. Reproduced with permission[262]. Copyright 2018 The Authors. Published by WILEY-VCH Verlag GmbH & Co. KGaA, Weinheim; (J) Wearable microneedle-based biosensor arrays for real-time sensing of alcohol and glucose in ISF. Reproduced with permission[263]. Copyright 2022, The Authors, under exclusive license to Springer Nature Limited; (K) Flexible biosensor for label-free detection of cortisol and glucose using non-faradaic electron-ionic charge transfer. Reproduced with permission[265]. Copyright 2016, Elsevier B.V. All rights reserved; (L) Wearable cortisol and glucose biosensor using monoclonal antibody-oriented approach. Reproduced with permission[266]. Copyright 2023, American Chemical Society; (M) A wearable electrochemical biosensor for monitoring of multiple metabolites and nutrients. Reproduced with permission[267]. Copyright 2022, The Author(s), under exclusive license to Springer Nature Limited. RE: Reference electrode; CE: counter electrode; PVA: poly(vinyl alcohol); ISF: interstitial fluid; β-HB: β-hydroxybutyrate.
DKA is considered as a severe complication of both type 1 and type 2 DM and still has high rates of mortality and morbidity[254]. β-Hydroxybutyrate (β-HB) is well known as a key factor for the diagnosis of DKA and a dominant biomarker of ketone formation. Because of this, many studies have been introduced to fulfill the demands for self-testing of ketone[255,256]. Despite major improvements in DM management, a real-time continuous ketone bodies monitoring (CKM) has not been as developed as CGM. Recently, Teymourian et al., from the group led by Wang, presented a microneedle-based device that enables the amperometric monitoring of HB and glucose, respectively [Figure 9E]. This CKM microneedle using HB dehydrogenase (HBD) enzymatic reaction solved the major challenges associated with the reliable confinement of the enzyme/cofactor couple (HBD/NAD+) and the fouling-free detection of nicotinamide adenine dinucleotide (NADH). The potential feasibility of CKM microneedles was demonstrated by showing an analytic performance, with good stability and high selectivity and sensitivity with a low detection limit of 50 μM in artificial ISF[257]. Furthermore, Moon et al. from the same group reported a touch-based sweat HB detection method, which relies on collecting fingertip sweat and transporting the sweat to a biocatalytic layer through a porous poly(vinyl alcohol) (PVA) hydrogel [Figure 9F]. The response on sweat HB was enhanced by mediating the oxidation reaction of NADH. A dual disposable biosensing device, integrating with ketone and glucose sensors, was demonstrated within healthy human subjects by simultaneously measuring the change of sweat HB and glucose levels during the intake of ketone supplements and glucose drinks[258].
Insulin is another significant biomarker for diagnosing DM, but the detection of insulin using a non-invasive wearable platform has some limitations due to extremely low concentrations in biofluids and interference from constituents in blood. Moreover, for detecting glucose and insulin simultaneously to improve the glycemic control and personalized health monitoring, a major challenge relies on integrating enzymatic and immunosensing electrochemical detection methods into a single system. Vargas et al., from the group led by Wang, addressed the different assay requirements by fabricating dual diabetes biomarker chip [Figure 9G]. Glucose detection was performed amperometrically through the biocatalytic oxidation of glucose, and insulin detection was conducted through the sandwich immunoreaction assay using horseradish peroxidase (HRP) as an enzymatic label and 3,3’,5,5’-tetramethylbenzidine (TMB)/H2O2 as the mediator/detection system. The glucose and insulin chip showed a considerable promise by accurately measuring two biomarkers with large concentration differences in blood and saliva[259]. Recently, Liu et al. developed an electrochemical aptasensor on carbon electrode, which enables real-time detection of glucose and insulin in saliva using two thiolated aptamers targeting glucose and insulin, respectively [Figure 9H]. The real-time detection was realized with a portable and wireless interface and demonstrated the sensing performance of the linearity in the range of 0.1-50 mM for glucose [limit of detection (LOD), 0.08 mM] and 0.05-15 nM for insulin (LOD, 0.85 nM)[260].
Cortisol is a glucocorticoid hormone that is significant for regulating glucose metabolism. In order to keep the glucose amounts in plasma, it increases the expression of glucogenesis-related enzymes. Moreover, according to recent studies, insufficient pancreatic insulin secretion may result from the disturbance in the circadian rhythm caused by cortisol[261]. Therefore, the simultaneous monitoring of glucose and cortisol can play an important role in better forecasting the onset of diabetes and/or stress-related symptoms. Munje
Alcohol and many other nutrients, such as amino acids and vitamins, can be additional factors for DM monitoring in terms of various food intake activities[264]. Kim et al., from the group led by Wang, presented a wearable device capable of measuring sweat-alcohol and ISF-glucose in human subjects while intaking food and drinking alcohol [Figure 9K]. This platform allowed on-demand sampling and analysis of two different biofluids, sweat and ISF, through sweat stimulation at an anode and ISF extraction at a cathode. The dual measurements of sweat-based alcohol and ISF-based glucose demonstrated good correlations of their blood levels[265]. With an advance of electronic systems and wearable technology, the same group developed wearable microneedle-based sensor arrays for real-time monitoring of various biomarkers, such as lactate and glucose, or alcohol and glucose in ISF biofluid [Figure 9L]. Reusability was enhanced using disposable MNA, electronics and optimized system integration. Through the comparison of gold-standard measurements in blood or breath, dual-analyte measurements using this system showed a good correlation with a commercial technology[266]. For more sophisticated metabolic profiling and monitoring, a universal wearable biosensing strategy using molecularly imprinted polymer (MIP)-based artificial antibodies was presented by Wang et al. [Figure 9M]. Autonomous and continuous molecular analysis was realized by incorporating iontophoresis-based sweat induction and microfluidic-based sweat sampling. Several human trials demonstrated the possibility of personalized monitoring of five essential or conditionally essential amino acids for central fatigue, standard dietary intake, and COVID-19 severity[267].
Despite these promising developments, effectively using wearable DM monitoring systems still requires more optimization and validation to thoroughly assess their reliability, versatility, and accuracy. These detailed studies will determine how well non-invasive methods can monitor biomarkers in biofluids and will compare the non-invasive data with traditional blood glucose measurements. Other limitations with wearable approaches include inconsistent collection of biofluids, contamination on the electrode surface, and the performance changes due to various physiological factors (such as temperature and pH) on the accuracy of the readings.
To enhance DM management, multiplexed analysis for the diabetes-related biomarkers, such as electrophysiological factors, insulin, ketone, alcohol, etc., can achieve a more comprehensive assessment of patient health than singular glucose measurement. Nevertheless, the implementation of multi-analyte sensing platforms entails several significant challenges, including the integration of different surface modifications and sensing modalities into a single wearable platform, the mitigation of analytical interference or cross-talk among different analytes, the necessity for receptor regeneration in bioaffinity-based assay methods, and the maximization of sensitivity for analytes in biofluids due to the lower concentration than blood. Consequently, future research should proceed in the direction of integrating glucose sensing with other novel analytes and skills for clinical use while overcoming these hurdles.
Machine learning-based multiplexed analysis for diabetes mellitus and its complications
In the evolving landscape of DM management, ML algorithms have emerged as a key technological advancement. Central to these algorithms is using extensive data sets to build predictive models, particularly vital in glucose monitoring applications. Wearable devices and CGM systems play a crucial role by providing the necessary input and output data. The process involves dividing this data into training and evaluation sets, which helps refine the model parameters and assess its performance. By harnessing sophisticated computational methodologies, there exists the prospect of substantially enhancing the management of DM, rendering it more individualized and attuned to the specific requirements of each patient. This entails the anticipation of prevailing glucose levels, prognostication of future trends, and the provision of informed recommendations for insulin administration. This section presents several ML techniques in glucose monitoring. The associated studies are summarized in [Table 7].
Machine learning-based multiplexed analysis for monitoring DM
Inputs | Aim | Data used | Algorithm | Performance | Ref. | |
1 | CGM | For forecasting glycemia | 25 T1DM patients for 14 days | ARIMA, RF and SVM | 15 min prediction horizon with RMSE of 11.65 mg/dL in just 16.15 s | [268] |
2 | Blood glucose | Hypoglycemia prediction | 200 samples (11 self-monitored blood glucose per sample) | RF, SVM, KNN and Naïve Bayes | Predicting a hypoglycemia event in the next 24 h with 92% sensitivity and 70% specificity | [269] |
3 | CGM | Predictive monitoring for glucose levels | 9 T1DM patients for 5 days | Data-driven autoregressive model | 30 min prediction horizon | [270] |
4 | CGM with meal information, insulin doses | Accurate glucose forecasting | 6 T1DM patients for 8 weeks | GluNet (CNN) | 19.2 mg/dL RMSE and 11.3 min time lag for 30 min prediction horizon | [271] |
5 | CGM, insulin, meal and physical activity | Continuous glucose monitoring using smartphones | Dataset collected from 112 participants (35 healthy, 38 with T1DM, and 39 with T2DM) | RNN | Achieved a blood glucose inference accuracy of 82.14% | [272] |
6 | Blood glucose, insulin doses, meal time and carbohydrate estimates | To make either insulin or carbohydrate recommendations | 12 subjects with T1DM from OhioT1DM dataset | 2 LSTM chain | 8.99 mg/dL RMSE for the carbohydrate recommendation | [273] |
7 | Glucose level, insulin bolus, and meal | For forecasting glucose levels | Dataset of 10 simulated cases and 10 clinical cases | Modified RNN with LSTM | RMSE of 9.38 and 18.87 mg/dL in simulated and clinical cases, respectively | [274] |
8 | CGM and exogenous events | Insulin bolus advisor | 10 adult subjects and 10 adolescent subjects from UVA/Padova T1D simulator | Deep reinforcement learning | Average percentage time of 80.9% and 61.6% in the adult and adolescent cohorts, respectively | [275] |
9 | 3 CGM (Abbott Diabetes, DexCom, Medtronic) | To predict the future glucose level of a new patient | Heterogeneous population of 451 T1DM patients | LSTM | Good prediction accuracy for both short-term (5.93 mg/dL RMSE, 30 min) and long-term (13.21 mg/dL RMSE, 60 min) prediction | [276] |
10 | Blood glucose | Accurate prediction of blood glucose variations in T2DM | 40 T2DM patients | Transfer learning | Over 95% prediction accuracy and 90% sensitivity for 1 h prediction horizon | [277] |
11 | Glucose, insulin and carbohydrate | Glucose predictor and patient classifier | 16 T1DM and 6 T2DM patients | CNN-based adversarial transfer learning | 19.92 mg/dL RMSE and 8.50 mg/dL MAPE | [278] |
12 | Sweat glucose | For measuring glucose concentrations from sweat | 3 human subjects | DT | RMSE of 0.1mg/dL | [279] |
13 | CGM, HR, steps, sleep and calories | To forecast the occurrence of hypoglycemia in the period when patients were sleeping | 10 T1DM patients | SVM and MLP | SVM achieved the best results in predicting nocturnal hypoglycemia | [280] |
Artificial neural network
Artificial neural networks (ANN) have become a cornerstone in advancing DM monitoring, primarily due to their ability to model complex relationships within data. The structure of an ANN is inspired by the human brain, comprising layers of interconnected nodes or neurons. These include input layers, which receive data such as blood glucose levels, dietary information and physical activity, hidden layers where the computation and pattern recognition occur, and an output layer that delivers the prediction or classification. The principle behind ANNs involves the neurons in each layer processing the input data and applying weights that are adjusted during training to minimize error in the output. This process, known as backpropagation, is critical for the ANN to learn from the data. As the network processes more data, it fine-tunes its weights, enhancing its predictive accuracy. In DM monitoring, various types of ANNs, such as recurrent neural networks (RNN), long short-term memory (LSTM) and convolutional neural networks (CNN), have been employed. Among them, Gu et al. presented the SugarMate, a smartphone-based blood glucose inference system, which offers a non-invasive alternative to CGM[272]. It integrated smartphone sensor data with food, drug, and insulin records to measure physical activity and sleep quality. Facing challenges of imbalanced and limited data, SugarMate employed Md3RNN, a deep RNN model with grouped input layers, for fine-grained blood glucose level inference. This model utilized limited personal and grouped-user data, achieving 82.14% accuracy in evaluations with 112 participants. Another recurrent convolutional neural network model was developed for precise blood glucose level forecasting in type 1 diabetes (T1D)[274]. The model achieves a root-mean-square error (RMSE) of 9.38 ± 0.71 mg/dL over 30 min and 18.87 ± 2.25 mg/dL over 60 min for simulated cases, and 21.07 ± 2.35 mg/dL for 30 min, 33.27% ± 4.79% for 60 min in real patient cases. In terms of LSTM-based models, Beauchamp et al. introduced a novel approach for managing blood glucose levels in T1D using a dual LSTM architecture[273]. This model inverted the traditional “what-if” scenario, instead providing recommendations for insulin dosages or carbohydrate intake to achieve target glucose levels. The technique was further enhanced by embedding the LSTM chain within a deep residual architecture, optimizing time series forecasting. This model demonstrated significant improvements for self-managing blood glucose levels in T1D over conventional methods by testing with real patient data. CNN was also used to forecast accurate glucose levels. Li et al. introduced GluNet, a personalized deep neural network framework for accurate short-term blood glucose prediction in T1D[271]. GluNet employed deep learning techniques and historical data, including glucose measurements, meals, and insulin doses. In simulations, it achieved a RMSE of 8.88 ± 0.77 mg/dL for 30-minute predictions and
Support vector machine
Support vector machines (SVM) are a type of supervised learning algorithm applicable to both classification and regression tasks. For classification, SVM distinguishes between two distinct classes by determining the most appropriate hyperplane that divides them, informed by the labeled training data. Depending on the task complexity and inter-feature relationships, SVM can employ either linear separation or more complex, non-linear methods. Non-linear classification challenges are managed by kernel functions that elevate the data into a higher-dimensional space, making the problem linearly separable. When it comes to regression, SVM aims to establish a function that lies within an acceptable deviation from the given targets, prioritizing the minimization of errors with a more flexible margin. This approach is similar to non-linear regression, wherein kernel functions are again leveraged to transform the data, thereby facilitating a linear approach to an intrinsically non-linear regression problem. For forecasting glycemia, CGM study from 25 DM type 1 patients was carried out in order to explore the viability of on-device computations with SVM[268]. This study found that a smartphone could predict glucose levels over a 15-minute horizon with a RMSE of
Decision tree
A decision tree (DT) is a method used to simplify data classification through a series of binary decisions, leading to a clear partition of data. It operates by splitting data at nodes based on attribute values until the data in each partition is sufficiently similar. DTs are utilized extensively in data mining and ML for regression and classification and are favored for their interpretability, offering valuable insights into key data attributes. For discrete target values, classification trees are utilized, while regression trees handle continuous ones. DTs can be adapted to various forms, including tree ensembles, to suit specific analytical needs and outcomes. One study introduced a non-invasive sweat sensor that measures glucose levels in eccrine sweat using electrochemical impedance spectroscopy[279]. With just 1-5 µL of sweat, the device provided real-time glucose measurements every few minutes. A ML algorithm, specifically DT regression, was used to process sensor data from three human subjects, achieving a high accuracy (R² of 0.94 and RMSE of 0.1 mg/dL).
Autoregressive integrated moving average
The autoregressive integrated moving average (ARIMA) model is a sophisticated linear method for forecasting future values in time series data, extending from past values. It integrates differencing operators with autoregressive and moving average components, making it a more generalized form of the autoregressive moving average (ARMA) model. The strength of ARIMA lies in its ability to accommodate a wide array of non-stationary series, thereby enhancing its applicability and accuracy in time series forecasting. A study monitored five DM patients for four days and forecasted glycemia by adapting ARIMA models with prediction horizons of 30 and 60 min, obtaining RMSEs of 22.9 and 42.2 mg/dL, respectively [281]. In addition, the ARIMA model was employed to forecast future trends in blood glucose levels, specifically targeting hypoglycemia and hyperglycemia[282]. This predictive framework was developed using CGM data from 100 patients, encompassing both T1D and type 2 diabetes (T2D). The model effectively generated early warnings, achieving a high sensitivity of 100% while maintaining a low false rate of 9.4%.
Transfer learning
Transfer learning is a ML technique where a model developed for one task is reused as the starting point for a model on a second task. This approach leverages pre-trained models to solve similar problems, significantly addressing the issue related to the need for a large amount of training data. It is particularly effective in deep learning, where substantial datasets and extensive training are typically required. Transfer learning is instrumental in various applications, including image and speech recognition, where it utilizes knowledge gained from one domain to improve learning in another, enhancing efficiency and performance in the learning process. Regarding DM monitoring, a study developed deep-learning methods for predicting blood glucose levels in T2D patients using continuous monitoring data, aiming to improve glycemic control and reduce complications[277]. The main challenges were small, imbalanced patient datasets and rare hypo/hyperglycemic events. By employing transfer learning and data augmentation, including techniques such as mix-up and generative models, the study achieved over 95% prediction accuracy and 90% sensitivity within a clinically relevant one-hour horizon. The approach was also effective for T1D, demonstrating its broader applicability. De Bois et al. also addressed the challenge of insufficient data in healthcare deep learning by proposing a multisource adversarial transfer learning framework[278]. This method enhanced data transfer across multiple health sources, learning a generalized feature representation. Applied to diabetic glucose forecasting, the approach showed improved statistical and clinical accuracies, particularly effective with diverse datasets or limited data scenarios. The adversarial transfer learning framework outperformed standard methods, promoting a more universal feature representation and improving deep model training with shared healthcare data.
For the advancement of DM monitoring, signal analysis using ML or deep learning techniques has been extensively researched for purposes such as forecasting glycemia, hypoglycemia, predictive monitoring for glucose levels, and insulin recommendation. Currently, these studies mainly utilize electrochemical signals derived from a single biomarker collected via invasive or minimally invasive methods, such as blood glucose or commercial CGM devices, as inputs for model training. Although there is research on applying the decision tree (DT) model to data non-invasively collected from wearable sweat-based glucose sensors to measure actual glucose concentration from sweat, this approach still faces limitations in continuous and predictive monitoring due to insufficient samples and unreliable concentration matching. For reliable, accurate, and timely monitoring of DM using wearable electrochemical sensors, developing new artificial intelligence (AI) models is essential. These models should be capable of matching the concentrations of biomarkers from various biofluids to their actual concentrations, quantitatively comparing the concentration differences between non-diabetics and diabetics, analyzing the correlations among multi-biomarkers, and examining the trends in concentrations according to the lifestyle and dietary habits of an individual.
CONCLUSIONS AND OUTLOOKS
In summary, this comprehensive review provides a thorough analysis of wearable sensor technology employing electrochemical methodologies for managing DM and its associated complications. The focal point is on developing non-invasive and minimally invasive electrochemical wearable sensors tailored for detecting diverse metabolites and electrolytes within human biofluids. The review encompasses a detailed exploration of electrochemical sensing mechanisms, the array of materials employed - including enzymes, non-enzyme assays, and polymer-based ISMs - and the diverse sensing modalities integrated into wearable electrochemical sensors. An in-depth examination of device architectures designed for monitoring specific metabolites and electrolytes in biofluids, such as sweat, tears, saliva, and ISF, is undertaken to augment the DM management efficacy. Furthermore, the review delves into wearable sensor devices engineered for a multiplexed monitoring platform, encompassing chemical-electrophysiological hybrid sensing systems and multiplexed electrochemical sensors capable of simultaneous monitoring of various biomarkers to enhance the DM management precision. The discourse extends to ML-based multiplexed analysis methodologies strategically devised for the proficient management of DM and its associated complications.
The escalating prevalence of DM has accelerated a growing reliance on glucose monitoring devices, necessitating the revision of care guidelines for diabetic individuals. Researchers have explored diverse diagnostic approaches beyond traditional glucose-related electrochemical reactions for DM management. Among these advancements are the incorporation of novel nanomaterial reaction pathways and enzyme electrodes and the merging of non-invasive or minimally invasive electrochemical sensor platforms with wearable bioelectronics and self-powered biosensors. While facing hurdles in advanced material technology and computational modeling, including accuracy, reliability, and environmental sustainability issues, endeavors are ongoing to create sensor systems with improved precision. Recognizing the limitations of single biomarker detection, there is a growing emphasis on multi-biomarker analysis technology. The convergence of technologies is emerging as a trend, facilitating the integration of different surface chemical compositions and detection principles into a single device. Techniques such as principal component analysis are employed to simplify the complexity of high-dimensional data, enhancing the maturity of detecting various combinations of biochemical indices and mitigating the impact of conflicting indicators on results. Future strategies for diabetes-related sensing prioritize improvements in cost-effectiveness, accuracy, precision, selectivity, and stability, aiming to provide more comfortable and secure conditions for patients. Advancements in miniaturization, portability, and enhanced software and hardware facilities have been made through various research studies [Table 8], and these advancements are anticipated in the flourishing field of electrochemical biosensing technology. Moreover, the adept arrangement of mobile devices and smartphone-oriented wireless platforms, integrating algorithmic applications, is positioned to streamline the deployment of wearable biosensors and affirm proof-of-concept demonstrations. This amalgamation of wearable biosensor technologies exhibits potential for augmenting human health and performance by enabling ongoing DM monitoring and interventions in the dynamic scope of daily life. Additionally, the incorporation of radiofrequency or Bluetooth-based identification, in conjunction with wearable electrochemical biosensors, is set to facilitate the effective data transmission from users to smartphones or computers. The forthcoming developments in wearable electrochemical biosensors are poised to facilitate the non-invasive monitoring of a diverse spectrum of biomarkers, spanning ions, drugs, toxins, metabolites, biomarkers, proteins, and immune assays. Furthermore, the development of closed-loop sensing and delivery systems emerges as a cornerstone of futuristic autonomous health management[283-285]. These systems, integrating real-time analysis with responsive drug delivery mechanisms, promise a paradigm shift towards personalized and precision medicine. By autonomously adjusting therapeutic interventions based on continuous biomarker monitoring, such systems aim to optimize patient healthcare, reduce the burden of disease management, and enhance the treatment efficacy. These advancements are anticipated to stimulate collaborative efforts across multiple disciplines, including nano-engineering, bioengineering, electronics, and medical communications.
Full system-level integration of wearable electrochemical sensors
Biofluid | Sampling strategy | Wearable configuration | Power source | Data communication | Ref. |
Sweat | Microfluidic channel | 3D printed watch using VEROBLACK and TANGOBLACK | Two batteries (3.7 V 155 mAh) | Measured using the Shimmer PCB and acquired with Consensys software | [210] |
Sweat | Microfluidic channel | Patch type | Rechargeable lithium-ion battery | Bluetooth module via UART protocol | [214] |
Sweat | Microfluidic channel | Patch type encapsulated by PDMS | Battery-free lactate biofuel cells | Wirelessly transmitted the data to the user interface using Bluetooth | [249] |
Sweat | Iontophoresis and microfluidic channel | Smartwatch integrated with sweat patch | Wire charging and rechargeable battery | Wirelessly transferred to the smartphone via Bluetooth | [267] |
Tear | Graphene channel | Contact lens encapsulated by elastofilcon A | Wireless power transfer (power transfer efficiency of 21.5% at the frequency of 50 MHz) | LED indicator | [224] |
Tear | Filter paper extraction | The miniatured system mounted on the eyeglasses frame | Rechargeable lithium-ion battery | BLE 5.1 | [226] |
Saliva | Oral | Mouthguard installed in oral cavity with PDMS sealing | Battery | BLE | [234] |
Saliva | PDMS-PEG microfluidic channel | Pacifier | Rechargeable battery with magnetic connector | BLE | [235] |
ISF | Microneedle | 3D printing supports (4 cm in diameter and 1.2 cm in thickness) | Rechargeable lithium-ion battery | Wirelessly transferred to the smartphone via Bluetooth | [242] |
ISF | Microneedle | 3D printing supports (2.6 cm in diameter) and disposable sensor | Wireless charging and rechargeable battery | BLE | [266] |
Despite these promising advancements for wearable DM monitoring systems, further research is still needed to optimize and validate their reliability, adaptability, and accuracy. In terms of non-invasive wearable approaches, various difficulties, such as, compared to traditional blood glucose measurement, uneven biofluid collection, surface contamination on the electrode, and variations in sensing performance brought on by different physiological parameters, remain as challenges to be resolved. Beyond single biomarker analysis, multiplexed analysis for diabetes-related biomarkers can offer a more thorough evaluation of patient health. However, a number of noteworthy obstacles are associated with deploying the multi-analyte sensing platform, including optimizing sensitivity for analytes in biofluids due to their lower concentration than blood, mitigating analytical interference or cross-talk among different analytes, integrating various surface modifications and sensing modalities into a single wearable platform, and requiring receptor regeneration in bioaffinity-based assay methods. AI can also be an effective solution for enhancing the DM monitoring performance. In order to play a better role beyond the existing performance such as forecasting glycemia, hypoglycemia, predictive monitoring for glucose levels, and insulin recommendation, new AI models should be able to compare quantitatively the concentration differences between individuals without diabetes and those with the disease, analyze correlations between multiple biomarkers, and look at trends in concentrations based on dietary and lifestyle choices using multi-analytes inputs. For clinical use of wearable electrochemical sensors, further efforts will be needed to address challenges such as concentration accuracy and response time delay that may occur in sweat or tears measurements, which include exploring clinical relevance compared to blood tests, multiplexed analysis, and advanced AI models capable of matching non-invasive data with gold-standard levels.
In conclusion, the remarkable progression of wearable electrochemical biosensor technology underscores its ability to furnish real-time quantitative and innovative information, particularly in the non-/minimally invasive management of DM. Notably, advancements in nanotechnology, precise data acquisition facilitated by nanotechnology, extensive data analysis through AI technology, and the incorporation of wireless technologies for point-of-care applications have the potential to revolutionize the healthcare management and diabetes prevention. In the realm of electrochemical sensors, developing wearable platforms has garnered considerable attention for their distinctive features. This is attributed to their capability to produce fully integrated devices in a compact, flexible, and robust manner, facilitating direct analysis of the human body and seamless wireless data transmission to a portable device. Continued research efforts will extend the range of sensing modalities, improving the accuracy and robustness of these systems for effective management and prevention of DM and its complications.
DECLARATIONS
Authors’ contributions
Outlined the manuscript structure: Jung HH, Lee H, Yea J
Conducted the literature review and wrote the manuscript: Jung HH, Lee H, Yea J
Reviewed and revised the manuscript: Jang KI, Jung HH
Availability of data and materials
Not applicable.
Financial support and sponsorship
This work was supported by the National Research Foundation of Korea (NRF) funded by the Ministry of Science and ICT (2020R1C1C1013030, 00234581) and Ministry of Trade, Industry and Energy (20010667). Further support was provided by the DGIST R&D Program of the Ministry of the Ministry of Science and ICT (24-SENS-01).
Conflict of interest
All authors declared that there are no conflicts of interest.
Ethical approval and consent to participate.
Not applicable.
Consent for publication
Not applicable.
Copyright
© The Author(s) 2024.
REFERENCES
1. Kaul K, Tarr JM, Ahmad SI, Kohner EM, Chibber R. Introduction to diabetes mellitus. Adv Exp Med Biol 2013;771:1-11.
2. International Diabetes Federation. IDF diabetes atlas 2021. Available from: https://diabetesatlas.org/atlas/tenth-edition/. [Last accessed on 15 Apr 2024].
3. NCD Risk Factor Collaboration (NCD-RisC). Worldwide trends in diabetes since 1980: a pooled analysis of 751 population-based studies with 4.4 million participants. Lancet 2016;387:1513-30.
4. Ortega Á, Berná G, Rojas A, Martín F, Soria B. Gene-diet interactions in type 2 diabetes: the chicken and egg debate. Int J Mol Sci 2017;18:1188.
5. Reddy SSK, Tan M. Chapter 1 - Diabetes mellitus and its many complications. In: Tan M, editor. Diabetes mellitus. Academic Press; 2020. pp. 1-18.
6. Lukovits TG, Mazzone TM, Gorelick TM. Diabetes mellitus and cerebrovascular disease. Neuroepidemiology 1999;18:1-14.
7. Ergul A, Kelly-Cobbs A, Abdalla M, Fagan SC. Cerebrovascular complications of diabetes: focus on stroke. Endocr Metab Immune Disord Drug Targets 2012;12:148-58.
8. Phipps MS, Jastreboff AM, Furie K, Kernan WN. The diagnosis and management of cerebrovascular disease in diabetes. Curr Diab Rep 2012;12:314-23.
9. Savage PJ. Cardiovascular complications of diabetes mellitus: what we know and what we need to know about their prevention. Ann Intern Med 1996;124:123-6.
10. Glovaci D, Fan W, Wong ND. Epidemiology of diabetes mellitus and cardiovascular disease. Curr Cardiol Rep 2019;21:21.
11. Smith SC Jr. Multiple risk factors for cardiovascular disease and diabetes mellitus. Am J Med 2007;120:S3-11.
12. Rivetti G, Hursh BE, Miraglia Del Giudice E, Marzuillo P. Acute and chronic kidney complications in children with type 1 diabetes mellitus. Pediatr Nephrol 2023;38:1449-58.
13. Hahr AJ, Molitch ME. Management of diabetes mellitus in patients with chronic kidney disease. Clin Diabetes Endocrinol 2015;1:2.
14. Alsahli M, Gerich JE. Hypoglycemia, chronic kidney disease, and diabetes mellitus. Mayo Clin Proc 2014;89:1564-71.
15. Cui Y, Andersen DK. Pancreatogenic diabetes: special considerations for management. Pancreatology 2011;11:279-94.
16. Bradley DA, Hart PA. Diabetes mellitus related to diseases of the exocrine pancreas (pancreatogenic diabetes). In: Domínguez-muñoz JE, editor. Clinical pancreatology for practising gastroenterologists and surgeons. Wiley; 2021. pp. 668-78.
18. Papatheodorou K, Banach M, Bekiari E, Rizzo M, Edmonds M. Complications of diabetes 2017. J Diabetes Res 2018; 2018:3086167.
19. Alberti KGMM, Zimmet PZ. Definition, diagnosis and classification of diabetes mellitus and its complications. Part 1: diagnosis and classification of diabetes mellitus provisional report of a WHO Consultation. Diabet Med 1998;15:539-53.
20. Unnikrishnan R, Anjana RM, Mohan V. Diabetes mellitus and its complications in India. Nat Rev Endocrinol 2016;12:357-70.
21. Harding JL, Pavkov ME, Magliano DJ, Shaw JE, Gregg EW. Global trends in diabetes complications: a review of current evidence. Diabetologia 2019;62:3-16.
22. World Health Organization. Global report on diabetes. 2016. Available from: https://www.who.int/publications/i/item/9789241565257. [Last accessed on 15 Apr 2024].
23. Centers for Disease Control and Prevention. Diabetes tests. 2023. Available from: https://www.cdc.gov/diabetes/basics/getting-tested.html. [Last accessed on 19 Apr 2024].
24. Santos Oliveira L, Oliveira SF, de Barros Manchado-Gobatto F, da Cunha Costa M. Salivary and blood lactate kinetics in response to maximal workload on cycle ergometer. Rev Bras Cineantropom Desempenho Hum 2015;17:565-74. (in Portuguese).
25. Aleksandar J, Vladan P, Markovic-Jovanovic S, Stolic R, Mitic J, Smilic T. Hyperlactatemia and the outcome of type 2 diabetic patients suffering acute myocardial infarction. J Diabetes Res 2016;2016:6901345.
26. D.S JP, Pasula S, Sunanda V, Apparow DN, kodali V. Study of uric acid and lipid profile in recent onset essential hypertension. IJCBR 2018;5:301-5. Available from: https://www.ijcbr.in/journal-article-file/6818. [Last accessed on 28 Apr 2024]
27. Wang Q, Wen X, Kong J. Recent progress on uric acid detection: a review. Crit Rev Anal Chem 2020;50:359-75.
28. Patterson MJ, Galloway SDR, Nimmo MA. Variations in regional sweat composition in normal human males. Exp Physiol 2000;85:869-75.
30. Sonner Z, Wilder E, Heikenfeld J, et al. The microfluidics of the eccrine sweat gland, including biomarker partitioning, transport, and biosensing implications. Biomicrofluidics 2015;9:031301.
31. Sato K, Kang WH, Saga K, Sato KT. Biology of sweat glands and their disorders. I. Normal sweat gland function. J Am Acad Dermatol 1989;20:537-63.
33. Xiao Y, Hou L, Wang M, et al. Noninvasive glucose monitoring using portable GOx-Based biosensing system. Anal Chim Acta 2024;1287:342068.
34. Gao W, Emaminejad S, Nyein HYY, et al. Fully integrated wearable sensor arrays for multiplexed in situ perspiration analysis. Nature 2016;529:509-14.
35. Emaminejad S, Gao W, Wu E, et al. Autonomous sweat extraction and analysis applied to cystic fibrosis and glucose monitoring using a fully integrated wearable platform. Proc Natl Acad Sci U S A 2017;114:4625-30.
36. Derbyshire PJ, Barr H, Davis F, Higson SP. Lactate in human sweat: a critical review of research to the present day. J Physiol Sci 2012;62:429-40.
37. Luo TT, Sun ZH, Li CX, Feng JL, Xiao ZX, Li WD. Monitor for lactate in perspiration. J Physiol Sci 2021;71:26.
38. Gupta S, Nayak MT, Sunitha JD, Dawar G, Sinha N, Rallan NS. Correlation of salivary glucose level with blood glucose level in diabetes mellitus. J Oral Maxillofac Pathol 2017;21:334-9.
39. Balan P, Babu SG, Sucheta KN, et al. Can saliva offer an advantage in monitoring of diabetes mellitus? - A case control study. J Clin Exp Dent 2014;6:e335-8.
40. Tékus E, Kaj M, Szabó E, et al. Comparison of blood and saliva lactate level after maximum intensity exercise. Acta Biol Hung 2012;63:89-98.
41. Zhao J, Huang Y. Salivary uric acid as a noninvasive biomarker for monitoring the efficacy of urate-lowering therapy in a patient with chronic gouty arthropathy. Clin Chim Acta 2015;450:115-20.
42. Singh G, Iyer EM, Malik H. Relative changes in salivary sodium and potassium in relation to exposure to high g stress. Med J Armed Forces India 1994;50:261-5.
43. Kallapur B, Ramalingam K, Bastian, Mujib A, Sarkar A, Sethuraman S. Quantitative estimation of sodium, potassium and total protein in saliva of diabetic smokers and nonsmokers: a novel study. J Nat Sci Biol Med 2013;4:341-5.
44. Sen DK, Sarin GS. Tear glucose levels in normal people and in diabetic patients. Br J Ophthalmol 1980;64:693-5.
45. Thomas N, Lähdesmäki I, Parviz B. A contact lens with an integrated lactate sensor. Sens Actuators B Chem 2012;162:128-34.
46. Horwath-Winter J, Kirchengast S, Meinitzer A, Wachswender C, Faschinger C, Schmut O. Determination of uric acid concentrations in human tear fluid, aqueous humour and serum. Acta Ophthalmol 2009;87:188-92.
47. Kim EH, Lee ES, Lee DY, Kim YP. Facile determination of sodium ion and osmolarity in artificial tears by sequential DNAzymes. Sensors 2017;17:2840.
48. Freckmann G, Hagenlocher S, Baumstark A, et al. Continuous glucose profiles in healthy subjects under everyday life conditions and after different meals. J Diabetes Sci Technol 2007;1:695-703.
49. Juvenile Diabetes Research Foundation Continuous Glucose Monitoring Study Group; Fox LA, Beck RW, Xing D. Variation of interstitial glucose measurements assessed by continuous glucose monitors in healthy, nondiabetic individuals. Diabetes Care 2010;33:1297-9.
50. Koschinsky T, Heinemann L. Sensors for glucose monitoring: technical and clinical aspects. Diabetes Metab Res Rev 2001;17:113-23.
51. Heikenfeld J, Jajack A, Feldman B, et al. Accessing analytes in biofluids for peripheral biochemical monitoring. Nat Biotechnol 2019;37:407-19.
52. Samant PP, Niedzwiecki MM, Raviele N, et al. Sampling interstitial fluid from human skin using a microneedle patch. Sci Transl Med 2020;12:eaaw0285.
53. Sieg A, Guy RH, Delgado-Charro MB. Noninvasive glucose monitoring by reverse iontophoresis in-vivo: application of the internal standard concept. Clin Chem 2004;50:1383-90.
54. Zacchia M, Abategiovanni ML, Stratigis S, Capasso G. Potassium: from physiology to clinical implications. Kidney Dis 2016;2:72-9.
55. Palmer BF, Clegg DJ. Physiology and pathophysiology of potassium homeostasis. Adv Physiol Educ 2016;40:480-90.
56. Xiong Q, Liu J, Xu Y. Effects of uric acid on diabetes mellitus and its chronic complications. Int J Endocrinol 2019;2019:9691345.
57. Woyesa SB, Gebisa WC, Anshebo DL. Assessment of selected serum electrolyte and associated risk factors in diabetic patients. Diabetes Metab Syndr Obes 2019;12:2811-7.
58. Klonoff DC, Ahn D, Drincic A. Continuous glucose monitoring: a review of the technology and clinical use. Diabetes Res Clin Pract 2017;133:178-92.
59. Mahato K, Wang J. Electrochemical sensors: from the bench to the skin. Sens Actuators B Chem 2021;344:130178.
60. Hernández-Rodríguez JF, Rojas D, Escarpa A. Electrochemical sensing directions for next-generation healthcare: trends, challenges, and frontiers. Anal Chem 2021;93:167-83.
61. Lee I, Probst D, Klonoff D, Sode K. Continuous glucose monitoring systems - current status and future perspectives of the flagship technologies in biosensor research. Biosens Bioelectron 2021;181:113054.
62. Zheng L, Zhu D, Wang W, Liu J, Thng STG, Chen P. A silk-microneedle patch to detect glucose in the interstitial fluid of skin or plant tissue. Sens Actuators B Chem 2022;372:132626.
63. Moonla C, Reynoso M, Casanova A, et al. Continuous ketone monitoring via wearable microneedle patch platform. ACS Sens 2024;9:1004-13.
64. Krentz AJ, Hompesch M. Glucose: archetypal biomarker in diabetes diagnosis, clinical management and research. Biomark Med 2016;10:1153-66.
65. Yan K, Zhang D, Wu D, Wei H, Lu G. Design of a breath analysis system for diabetes screening and blood glucose level prediction. IEEE Trans Biomed Eng 2014;61:2787-95.
66. Anders HJ, Huber TB, Isermann B, Schiffer M. CKD in diabetes: diabetic kidney disease versus nondiabetic kidney disease. Nat Rev Nephrol 2018;14:361-77.
67. Hatada M, Wilson E, Khanwalker M, Probst D, Okuda-shimazaki J, Sode K. Current and future prospective of biosensing molecules for point-of-care sensors for diabetes biomarker. Sens Actuators B Chem 2022;351:130914.
68. Crawford SO, Hoogeveen RC, Brancati FL, et al. Association of blood lactate with type 2 diabetes: the Atherosclerosis Risk in Communities Carotid MRI Study. Int J Epidemiol 2010;39:1647-55.
69. Azushima K, Kovalik JP, Yamaji T, et al. Abnormal lactate metabolism is linked to albuminuria and kidney injury in diabetic nephropathy. Kidney Int 2023;104:1135-49.
70. Pecoits-Filho R, Abensur H, Betônico CC, et al. Interactions between kidney disease and diabetes: dangerous liaisons. Diabetol Metab Syndr 2016;8:50.
71. Baruh S, Sherman L, Markowitz S. Diabetic ketoacidosis and coma. Med Clin North Am 1981;65:117-32.
73. Beigelman PM. Severe diabetic ketoacidosis (diabetic “coma”). 482 episodes in 257 patients; experience of three years. Diabetes 1971;20:490-500.
74. Lytvyn Y, Perkins BA, Cherney DZ. Uric acid as a biomarker and a therapeutic target in diabetes. Can J Diabetes 2015;39:239-46.
75. Heerspink HJ, Perkins BA, Fitchett DH, Husain M, Cherney DZ. Sodium glucose cotransporter 2 inhibitors in the treatment of diabetes mellitus: cardiovascular and kidney effects, potential mechanisms, and clinical applications. Circulation 2016;134:752-72.
76. Din UAAS, Salem MM, Abdulazim DO. Uric acid in the pathogenesis of metabolic, renal, and cardiovascular diseases: a review. J Adv Res 2017;8:537-48.
77. Mou L, Xia Y, Jiang X. Epidermal sensor for potentiometric analysis of metabolite and electrolyte. Anal Chem 2021;93:11525-31.
78. Liamis G, Liberopoulos E, Barkas F, Elisaf M. Diabetes mellitus and electrolyte disorders. World J Clin Cases 2014;2:488-96.
79. Mirzajani H, Mirlou F, Istif E, Singh R, Beker L. Powering smart contact lenses for continuous health monitoring: Recent advancements and future challenges. Biosens Bioelectron 2022;197:113761.
80. Liu H, Yan X, Gu Z, Xiu G, Xiao X. Electrochemical sensing in contact lenses. Electroanalysis 2022;34:227-36.
81. Kim J, Cha E, Park JU. Recent advances in smart contact lenses. Adv Mater Technol 2020;5:1900728.
82. Zhu Y, Li S, Li J, et al. Lab-on-a-contact lens: recent advances and future opportunities in diagnostics and therapeutics. Adv Mater 2022;34:e2108389.
83. Jin X, Cai A, Xu T, Zhang X. Artificial intelligence biosensors for continuous glucose monitoring. Interdiscip Mater 2023;2:290-307.
84. Das SK, Nayak KK, Krishnaswamy PR, Kumar V, Bhat N. Review - electrochemistry and other emerging technologies for continuous glucose monitoring devices. ECS Sens Plus 2022;1:031601.
86. Guinovart T, Parrilla M, Crespo GA, Rius FX, Andrade FJ. Potentiometric sensors using cotton yarns, carbon nanotubes and polymeric membranes. Analyst 2013;138:5208-15.
87. Parrilla M, Ferré J, Guinovart T, Andrade FJ. Wearable potentiometric sensors based on commercial carbon fibres for monitoring sodium in sweat. Electroanalysis 2016;28:1267-75.
88. Jung HH, Yea J, Lee H, et al. Taste bud-inspired single-drop multitaste sensing for comprehensive flavor analysis with deep learning algorithms. ACS Appl Mater Interfaces 2023;15:46041-53.
89. Jung HH, Ha J, Park J, et al. Highly deformable double-sided neural probe with all-in-one electrode system for real-time in-vivo detection of dopamine for Parkinson’s disease. Adv Funct Mater 2024;34:2311436.
90. Liu G, Lin Y. Amperometric glucose biosensor based on self-assembling glucose oxidase on carbon nanotubes. Electrochem Commun 2006;8:251-6.
91. Romero MR, Ahumada F, Garay F, Baruzzi AM. Amperometric biosensor for direct blood lactate detection. Anal Chem 2010;82:5568-72.
92. Vidya H, Kumara Swamy BE, Sharma SC, Jayaprakash GK, Hariprasad SA. Effect of graphite oxide and exfoliated graphite oxide as a modifier for the voltametric determination of dopamine in presence of uric acid and folic acid. Sci Rep 2021;11:24040.
93. Alimohammadi S, Kiani MA, Imani M, Rafii-Tabar H, Sasanpour P. A proposed implantable voltammetric carbon fiber-based microsensor for corticosteroid monitoring by cochlear implants. Mikrochim Acta 2021;188:357.
94. Lei Y, Butler D, Lucking MC, et al. Single-atom doping of MoS2 with manganese enables ultrasensitive detection of dopamine: experimental and computational approach. Sci Adv 2020;6:eabc4250.
95. Khan RN, Saba F, Kausar SF, Siddiqui MH. Pattern of electrolyte imbalance in Type 2 diabetes patients: experience from a tertiary care hospital. Pak J Med Sci 2019;35:797-801.
96. Campuzano S, Pedrero M, Torrente-rodríguez RM, Pingarrón JM. Affinity-based wearable electrochemical biosensors: natural versus biomimetic receptors. Anal Sens 2023;3:e202200087.
97. García-Salas JM, Tello-Montoliu A, Manzano-Fernández S, et al. Interleukin-6 as a predictor of cardiovascular events in troponin-negative non-ST elevation acute coronary syndrome patients. Int J Clin Pract 2014;68:294-303.
98. Ferreira PC, Ataíde VN, Silva Chagas CL, et al. Wearable electrochemical sensors for forensic and clinical applications. TrAC Trends Anal Chem 2019;119:115622.
99. Yang Y, Feijóo J, Briega-martos V, et al. Operando methods: a new era of electrochemistry. Curr Opin Electrochem 2023;42:101403.
101. Lee H, Hong YJ, Baik S, Hyeon T, Kim DH. Enzyme-based glucose sensor: from invasive to wearable device. Adv Healthc Mater 2018;7:e1701150.
102. Bollella P, Sharma S, Cass AEG, Antiochia R. Microneedle-based biosensor for minimally-invasive lactate detection. Biosens Bioelectron 2019;123:152-9.
103. Sun M, Cui C, Chen H, Wang D, Zhang W, Guo W. Enzymatic and non-enzymatic uric acid electrochemical biosensors: a review. Chempluschem 2023;88:e202300262.
104. Rocchitta G, Secchi O, Alvau MD, et al. Development and characterization of an implantable biosensor for telemetric monitoring of ethanol in the brain of freely moving rats. Anal Chem 2012;84:7072-9.
105. Rasitanon N, Ittisoponpisan S, Kaewpradub K, Jeerapan I. Wearable electrodes for lactate: applications in enzyme-based sensors and energy biodevices. Anal Sens 2023;3:e202200066.
106. Harper A, Anderson MR. Electrochemical glucose sensors - developments using electrostatic assembly and carbon nanotubes for biosensor construction. Sensors 2010;10:8248-74.
107. Ghindilis AL, Krishnan R, Atanasov P, Wilkins E. Flow-through amperometric immunosensor: fast ‘sandwich’ scheme immunoassay. Biosens Bioelectron 1997;12:415-23.
108. Antiochia R, Cass AEG, Palleschi G. Purification and sensor applications of an oxygen insensitive, thermophilic diaphorase. Anal Chim Acta 1997;345:17-28.
110. Bridge JA, Iyengar S, Salary CB, et al. Clinical response and risk for reported suicidal ideation and suicide attempts in pediatric antidepressant treatment: a meta-analysis of randomized controlled trials. JAMA 2007;297:1683-96.
111. Ghindilis AL, Atanasov P, Wilkins E. Enzyme-catalyzed direct electron transfer: fundamentals and analytical applications. Electroanalysis 1997;9:661-74.
112. Gorton L. Carbon paste electrodes modified with enzymes, tissues, and cells. Electroanalysis 1995;7:23-45.
113. Gorton L, Lindgren A, Larsson T, Munteanu F, Ruzgas T, Gazaryan I. Direct electron transfer between heme-containing enzymes and electrodes as basis for third generation biosensors. Anal Chim Acta 1999;400:91-108.
114. Zhang W, Li G. Third-generation biosensors based on the direct electron transfer of proteins. Anal Sci 2004;20:603-9.
115. Xiao X, Xia HQ, Wu R, et al. Tackling the challenges of enzymatic (bio)fuel cells. Chem Rev 2019;119:9509-58.
116. Wilson JR, Caruana DJ, Gilardi G. Engineering redox functions in a nucleic acid binding protein. Chem Commun 2003:356-7.
117. Zayats M, Katz E, Willner I. Electrical contacting of glucose oxidase by surface-reconstitution of the apo-protein on a relay-boronic acid-FAD cofactor monolayer. J Am Chem Soc 2002;124:2120-1.
118. Jesionowski T, Zdarta J, Krajewska B. Enzyme immobilization by adsorption: a review. Adsorption 2014;20:801-21.
119. Imam HT, Marr PC, Marr AC. Enzyme entrapment, biocatalyst immobilization without covalent attachment. Green Chem 2021;23:4980-5005.
120. Zucca P, Sanjust E. Inorganic materials as supports for covalent enzyme immobilization: methods and mechanisms. Molecules 2014;19:14139-94.
121. Harris JM, Reyes C, Lopez GP. Common causes of glucose oxidase instability in in-vivo biosensing: a brief review. J Diabetes Sci Technol 2013;7:1030-8.
122. Guzsvány V, Anojčić J, Radulović E, et al. Screen-printed enzymatic glucose biosensor based on a composite made from multiwalled carbon nanotubes and palladium containing particles. Microchim Acta 2017;184:1987-96.
123. Sanaeifar N, Rabiee M, Abdolrahim M, Tahriri M, Vashaee D, Tayebi L. A novel electrochemical biosensor based on Fe3O4 nanoparticles-polyvinyl alcohol composite for sensitive detection of glucose. Anal Biochem 2017;519:19-26.
124. Baby TT, Ramaprabhu S. SiO2 coated Fe3O4 magnetic nanoparticle dispersed multiwalled carbon nanotubes based amperometric glucose biosensor. Talanta 2010;80:2016-22.
125. Shukla M, Pramila, Dixit T, Prakash R, Palani IA, Singh V. Influence of aspect ratio and surface defect density on hydrothermally grown ZnO nanorods towards amperometric glucose biosensing applications. Appl Surf Sci 2017;422:798-808.
126. Mohamad NR, Marzuki NHC, Buang NA, Huyop F, Wahab RA. An overview of technologies for immobilization of enzymes and surface analysis techniques for immobilized enzymes. Biotechnol Biotechnol Equip 2015;29:205-20.
127. Ichimura K, Watanabe S. Preparation and characteristics of photocross-linkable poly(vinyl alcohol). J Polym Sci Polym Chem Ed 1982;20:1419-32.
128. Ichimura K. A convenient photochemical method to immobilize enzymes. J Polym Sci Polym Chem Ed 1984;22:2817-28.
129. Kandimalla VB, Tripathi VS, Ju H. Immobilization of biomolecules in sol-gels: biological and analytical applications. Crit Rev Anal Chem 2006;36:73-106.
130. Gupta R, Chaudhury NK. Entrapment of biomolecules in sol-gel matrix for applications in biosensors: problems and future prospects. Biosens Bioelectron 2007;22:2387-99.
132. King GA, Daugulis AJ, Faulkner P, Goosen MFA. Alginate-polylysine microcapsules of controlled membrane molecular weight cutoff for mammalian cell culture engineering. Biotechnol Prog 1987;3:231-40.
133. Nguyen HH, Kim M. An overview of techniques in enzyme immobilization. Appl Sci Converg Technol 2017;26:157-63.
134. Gustafsson H, Thörn C, Holmberg K. A comparison of lipase and trypsin encapsulated in mesoporous materials with varying pore sizes and pH conditions. Colloids Surf B Biointerfaces 2011;87:464-71.
135. Matsuura S, Ishii R, Itoh T, et al. Immobilization of enzyme-encapsulated nanoporous material in a microreactor and reaction analysis. Chem Eng J 2011;167:744-9.
136. Chakraborty S, Rusli H, Nath A, et al. Immobilized biocatalytic process development and potential application in membrane separation: a review. Crit Rev Biotechnol 2016;36:43-58.
137. Sulaiman S, Mokhtar MN, Naim MN, Baharuddin AS, Sulaiman A. A review: potential usage of cellulose nanofibers (CNF) for enzyme immobilization via covalent interactions. Appl Biochem Biotechnol 2015;175:1817-42.
138. Marrazza G. Piezoelectric biosensors for organophosphate and carbamate pesticides: a review. Biosensors 2014;4:301-17.
139. Regiart M, Ledo A, Fernandes E, et al. Highly sensitive and selective nanostructured microbiosensors for glucose and lactate simultaneous measurements in blood serum and in-vivo in brain tissue. Biosens Bioelectron 2022;199:113874.
140. Narwal V, Sharma M, Rani S, Pundir CS. An ultrasensitive amperometric determination of lactate by lactate dehydrogenase nanoparticles immobilized onto Au electrode. Int J Biol Macromol 2018;115:767-75.
141. Dasgupta A, Sori N, Petrova S, et al. Comprehensive collagen crosslinking comparison of microfluidic wet-extruded microfibers for bioactive surgical suture development. Acta Biomater 2021;128:186-200.
142. Mir AR, Habib S, Uddin M. Recent advances in histone glycation: emerging role in diabetes and cancer. Glycobiology 2021;31:1072-9.
143. Sonker AK, Belay M, Rathore K, et al. Crosslinking of agar by diisocyanates. Carbohydr Polym 2018;202:454-60.
144. Feng R, Chu Y, Wang X, Wu Q, Tang F. A long-term stable and flexible glucose sensor coated with poly(ethylene glycol)-modified polyurethane. J Electroanal Chem 2021;895:115518.
145. Chang BS, Mahoney RR. Enzyme thermostabilization by bovine serum albumin and other proteins: evidence for hydrophobic interactions. Biotechol Appl Biochem 1995;22:203-14.
147. Das S, Mishra B, Gill K, et al. Isolation and characterization of novel protein with anti-fungal and anti-inflammatory properties from Aloe vera leaf gel. Int J Biol Macromol 2011;48:38-43.
148. Fopase R, Paramasivam S, Kale P, Paramasivan B. Strategies, challenges and opportunities of enzyme immobilization on porous silicon for biosensing applications. J Environ Chem Eng 2020;8:104266.
149. Perez JJ, Francois NJ, Maroniche GA, Borrajo MP, Pereyra MA, Creus CM. A novel, green, low-cost chitosan-starch hydrogel as potential delivery system for plant growth-promoting bacteria. Carbohydr Polym 2018;202:409-17.
150. Shao Y, Wan H, Miao J, Guan G. Synthesis of an immobilized Brønsted acidic ionic liquid catalyst on chloromethyl polystyrene grafted silica gel for esterification. Reac Kinet Mech Cat 2013;109:149-58.
152. Tang ZM, Kang JW. Enzyme inhibitor screening by capillary electrophoresis with an on-column immobilized enzyme microreactor created by an ionic binding technique. Anal Chem 2006;78:2514-20.
153. Hwang ET, Gu MB. Enzyme stabilization by nano/microsized hybrid materials. Eng Life Sci 2013;13:49-61.
154. Kaur J, Choudhary S, Chaudhari R, Jayant RD, Joshi A. 9 - Enzyme-based biosensors. In: Pal K, Kraatz HB, Khasnobish A, Bag S, Banerjee I, Kuruganti U, editors. Bioelectronics and medical devices. Woodhead Publishing; 2019. pp. 211-40.
155. Sirisha VL, Jain A, Jain A. Chapter nine - Enzyme immobilization: an overview on methods, support material, and applications of immobilized enzymes. Adv Food Nutr Res 2016;79:179-211.
156. Moreno-Bondi MC, Taitt CR, Shriver-Lake LC, Ligler FS. Multiplexed measurement of serum antibodies using an array biosensor. Biosens Bioelectron 2006;21:1880-6.
157. Akter R, Jeong B, Lee YM, Choi JS, Rahman MA. Femtomolar detection of cardiac troponin I using a novel label-free and reagent-free dendrimer enhanced impedimetric immunosensor. Biosens Bioelectron 2017;91:637-43.
158. Andoy NM, Filipiak MS, Vetter D, Gutiérrez-sanz Ó, Tarasov A. Graphene-based electronic immunosensor with femtomolar detection limit in whole serum. Adv Mater Technol 2018;3:1800186.
159. Basu J, Datta S, RoyChaudhuri C. A graphene field effect capacitive Immunosensor for sub-femtomolar food toxin detection. Biosens Bioelectron 2015;68:544-9.
160. Wehmeyer KR, White RJ, Kissinger PT, Heineman WR. Electrochemical affinity assays/sensors: brief history and current status. Annu Rev Anal Chem 2021;14:109-31.
161. Tu J, Torrente-Rodríguez RM, Wang M, Gao W. The era of digital health: a review of portable and wearable affinity biosensors. Adv Funct Mater 2020;30:1906713.
162. Sempionatto JR, Lasalde-Ramírez JA, Mahato K, Wang J, Gao W. Wearable chemical sensors for biomarker discovery in the omics era. Nat Rev Chem 2022;6:899-915.
163. Flynn CD, Chang D, Mahmud A, et al. Biomolecular sensors for advanced physiological monitoring. Nat Rev Bioeng 2023;1:560-75.
164. Guo W, Zhang C, Ma T, et al. Advances in aptamer screening and aptasensors’ detection of heavy metal ions. J Nanobiotechnology 2021;19:166.
165. Yang D, Liu X, Zhou Y, et al. Aptamer-based biosensors for detection of lead(ii) ion: a review. Anal Methods 2017;9:1976-90.
166. Wang W, Chen C, Qian M, Zhao XS. Aptamer biosensor for protein detection using gold nanoparticles. Anal Biochem 2008;373:213-9.
167. Cheng AK, Sen D, Yu HZ. Design and testing of aptamer-based electrochemical biosensors for proteins and small molecules. Bioelectrochemistry 2009;77:1-12.
168. Frutiger A, Tanno A, Hwu S, Tiefenauer RF, Vörös J, Nakatsuka N. Nonspecific binding-fundamental concepts and consequences for biosensing applications. Chem Rev 2021;121:8095-160.
169. Blind M, Blank M. Aptamer selection technology and recent advances. Mol Ther Nucleic Acids 2015;4:e223.
170. Kohlberger M, Gadermaier G. SELEX: Critical factors and optimization strategies for successful aptamer selection. Biotechnol Appl Biochem 2022;69:1771-92.
171. Lyu C, Khan IM, Wang Z. Capture-SELEX for aptamer selection: a short review. Talanta 2021;229:122274.
172. Yano-Ozawa Y, Lobsiger N, Muto Y, et al. Molecular detection using aptamer-modified gold nanoparticles with an immobilized DNA brush for the prevention of non-specific aggregation. RSC Adv 2021;11:11984-91.
173. Liu Y, Canoura J, Alkhamis O, Xiao Y. Immobilization strategies for enhancing sensitivity of electrochemical aptamer-based sensors. ACS Appl Mater Interfaces 2021;13:9491-9.
174. Oberhaus FV, Frense D, Beckmann D. Immobilization techniques for aptamers on gold electrodes for the electrochemical detection of proteins: a review. Biosensors 2020;10:45.
175. Herrera-Chacón A, Cetó X, Del Valle M. Molecularly imprinted polymers - towards electrochemical sensors and electronic tongues. Anal Bioanal Chem 2021;413:6117-40.
176. Gui R, Jin H, Guo H, Wang Z. Recent advances and future prospects in molecularly imprinted polymers-based electrochemical biosensors. Biosens Bioelectron 2018;100:56-70.
177. Lowdon JW, Diliën H, Singla P, et al. MIPs for commercial application in low-cost sensors and assays - an overview of the current status quo. Sens Actuators B Chem 2020;325:128973.
178. Denmark DJ, Mohapatra S, Mohapatra SS. Point-of-care diagnostics: molecularly imprinted polymers and nanomaterials for enhanced biosensor selectivity and transduction. EuroBiotech J 2020;4:184-206.
179. Pourmadadi M, Soleimani Dinani H, Saeidi Tabar F, et al. Properties and applications of graphene and its derivatives in biosensors for cancer detection: a comprehensive review. Biosensors 2022;12:269.
180. Morales MA, Halpern JM. Guide to Selecting a Biorecognition element for biosensors. Bioconjug Chem 2018;29:3231-9.
181. Choi KR, Troudt BK, Bühlmann P. Ion-selective electrodes with sensing membranes covalently attached to both the inert polymer substrate and conductive carbon contact. Angew Chem Int Ed Engl 2023;62:e202304674.
182. De Marco R, Clarke G, Pejcic B. Ion-selective electrode potentiometry in environmental analysis. Electroanalysis 2007;19:1987-2001.
183. Ding J, Qin W. Recent advances in potentiometric biosensors. TrAC Trends Anal Chem 2020;124:115803.
185. Cuartero M, Parrilla M, Crespo GA. Wearable potentiometric sensors for medical applications. Sensors 2019;19:363.
186. Lyu Y, Gan S, Bao Y, et al. Solid-contact ion-selective electrodes: response mechanisms, transducer materials and wearable sensors. Membranes 2020;10:128.
188. Bakker E, Nägele M, Schaller U, Pretsch E. Applicability of the phase boundary potential model to the mechanistic understanding of solvent polymeric membrane-based ion-selective electrodes. Electroanalysis 1995;7:817-22.
189. Bakker E, Chumbimuni-Torres K. Modern directions for potentiometric sensors. J Braz Chem Soc 2008;19:621-9.
190. Shao Y, Ying Y, Ping J. Recent advances in solid-contact ion-selective electrodes: functional materials, transduction mechanisms, and development trends. Chem Soc Rev 2020;49:4405-65.
191. Janata J, Josowicz M. Nernstian and non-nernstian potentiometry. Solid State Ionics 1997;94:209-15.
192. Migdalski J, Lewenstam A. Electrically enhanced sensitivity (EES) of ion-selective membrane electrodes and membrane-based ion sensors. Membranes 2022;12:763.
193. Amemiya S, Bühlmann P, Odashima K. A generalized model for apparently “non-Nernstian” equilibrium responses of ionophore-based ion-selective electrodes. 1. Independent complexation of the ionophore with primary and secondary ions. Anal Chem 2003;75:3329-39.
194. Jackson DT, Nelson PN. Preparation and properties of some ion selective membranes: a review. JMol Struct 2019;1182:241-59.
195. Hu J, Stein A, Bühlmann P. Rational design of all-solid-state ion-selective electrodes and reference electrodes. TrAC Trends Anal Chem 2016;76:102-14.
196. Valeri C, Pozzilli P, Leslie D. Glucose control in diabetes. Diabetes Metab Res Rev 2004;20:S1-8.
197. Musen G, Jacobson AM, Ryan CM, et al. Diabetes Control and Complications Trial/Epidemiology of Diabetes Interventions and Complications Research Group. Glycemic control and hypoglycemia: is the loser the winner? Response to Perlmuter et al. Diabetes Care 2009;32:e46.
198. Militaru A, Frandes M, Lungeanu D. Smart wristbands as inexpensive and reliable non-dedicated solution for self-managing type 2 diabetes. In: 2015 E-Health and Bioengineering Conference (EHB); 2015 Nov 19-21; Iasi, Romania. IEEE; 2015. pp. 1-4.
199. Aslam MW, Zhu Z, Nandi AK. Feature generation using genetic programming with comparative partner selection for diabetes classification. Expert Syst Appl 2013;40:5402-12.
200. Ozana N, Beiderman Y, Anand A, et al. Noncontact speckle-based optical sensor for detection of glucose concentration using magneto-optic effect. J Biomed Opt 2016;21:65001.
201. Acharya U, Faust O, Adib Kadri N, Suri JS, Yu W. Automated identification of normal and diabetes heart rate signals using nonlinear measures. Comput Biol Med 2013;43:1523-9.
202. Zafar H, Channa A, Jeoti V, Stojanović GM. Comprehensive review on wearable sweat-glucose sensors for continuous glucose monitoring. Sensors 2022;22:638.
203. Moyer J, Wilson D, Finkelshtein I, Wong B, Potts R. Correlation between sweat glucose and blood glucose in subjects with diabetes. Diabetes Technol Ther 2012;14:398-402.
204. Pullano SA, Greco M, Bianco MG, Foti D, Brunetti A, Fiorillo AS. Glucose biosensors in clinical practice: principles, limits and perspectives of currently used devices. Theranostics 2022;12:493-511.
205. Aihara M, Kubota N, Kadowaki T. Study of the correlation between tear glucose concentrations and blood glucose concentrations. Diabetes 2018;67:944-P.
206. Agrawal RP, Sharma N, Rathore MS, et al. Noninvasive method for glucose level estimation by saliva. J Diabetes Metab 2013;4:266. Available from: https://www.researchgate.net/profile/Vivek-Agarwal-13/publication/337591632_Noninvasive_Method_for_Glucose_Level_Estimation_by_Saliva/links/5ddf733aa6fdcc2837f05fb9/Noninvasive-Method-for-Glucose-Level-Estimation-by-Saliva.pdf.[Last accessed on 28 Apr 2024]
207. Zhang J, Xu J, Lim J, Nolan JK, Lee H, Lee CH. Wearable glucose monitoring and implantable drug delivery systems for diabetes management. Adv Healthc Mater 2021;10:e2100194.
208. Grieshaber D, MacKenzie R, Vörös J, Reimhult E. Electrochemical biosensors - sensor principles and architectures. Sensors 2008;8:1400-58.
209. Mohan A, Rajendran V, Mishra RK, Jayaraman M. Recent advances and perspectives in sweat based wearable electrochemical sensors. TrAC Trends Anal Chem 2020;131:116024.
210. Pirovano P, Dorrian M, Shinde A, et al. A wearable sensor for the detection of sodium and potassium in human sweat during exercise. Talanta 2020;219:121145.
211. Zhao C, Li X, Wu Q, Liu X. A thread-based wearable sweat nanobiosensor. Biosens Bioelectron 2021;188:113270.
212. Wang S, Wu Y, Gu Y, et al. Wearable sweatband sensor platform based on gold nanodendrite array as efficient solid contact of ion-selective electrode. Anal Chem 2017;89:10224-31.
213. Jeerapan I, Sempionatto JR, Pavinatto A, You JM, Wang J. Stretchable biofuel cells as wearable textile-based self-powered sensors. J Mater Chem A Mater 2016;4:18342-53.
214. Nyein HYY, Bariya M, Kivimäki L, et al. Regional and correlative sweat analysis using high-throughput microfluidic sensing patches toward decoding sweat. Sci Adv 2019;5:eaaw9906.
215. Chen C, Dong ZQ, Shen JH, Chen HW, Zhu YH, Zhu ZG. 2D photonic crystal hydrogel sensor for tear glucose monitoring. ACS Omega 2018;3:3211-7.
216. Alexeev VL, Das S, Finegold DN, Asher SA. Photonic crystal glucose-sensing material for noninvasive monitoring of glucose in tear fluid. Clin Chem 2004;50:2353-60.
217. Jeon HJ, Kim S, Park S, et al. Optical assessment of tear glucose by smart biosensor based on nanoparticle embedded contact lens. Nano Lett 2021;21:8933-40.
218. Fang H, Kaur G, Wang B. Progress in boronic acid-based fluorescent glucose sensors. J Fluoresc 2004;14:481-9.
219. Chen L, Tse WH, Chen Y, McDonald MW, Melling J, Zhang J. Nanostructured biosensor for detecting glucose in tear by applying fluorescence resonance energy transfer quenching mechanism. Biosens Bioelectron 2017;91:393-9.
220. Yan Q, Peng B, Su G, Cohan BE, Major TC, Meyerhoff ME. Measurement of tear glucose levels with amperometric glucose biosensor/capillary tube configuration. Anal Chem 2011;83:8341-6.
221. Chu MX, Miyajima K, Takahashi D, et al. Soft contact lens biosensor for in situ monitoring of tear glucose as non-invasive blood sugar assessment. Talanta 2011;83:960-5.
222. Yao H, Shum AJ, Cowan M, Lähdesmäki I, Parviz BA. A contact lens with embedded sensor for monitoring tear glucose level. Biosens Bioelectron 2011;26:3290-6.
223. Kim J, Kim M, Lee MS, et al. Wearable smart sensor systems integrated on soft contact lenses for wireless ocular diagnostics. Nat Commun 2017;8:14997.
224. Park J, Kim J, Kim SY, et al. Soft, smart contact lenses with integrations of wireless circuits, glucose sensors, and displays. Sci Adv 2018;4:eaap9841.
225. Keum DH, Kim SK, Koo J, et al. Wireless smart contact lens for diabetic diagnosis and therapy. Sci Adv 2020;6:eaba3252.
226. Sempionatto JR, Brazaca LC, García-Carmona L, et al. Eyeglasses-based tear biosensing system: Non-invasive detection of alcohol, vitamins and glucose. Biosens Bioelectron 2019;137:161-70.
227. Matzeu G, Florea L, Diamond D. Advances in wearable chemical sensor design for monitoring biological fluids. Sens Actuators B Chem 2015;211:403-18.
228. Goldoni R, Scolaro A, Boccalari E, et al. Malignancies and biosensors: a focus on oral cancer detection through salivary biomarkers. Biosensors 2021;11:396.
229. Bel’skaya LV, Sarf EA, Makarova NA. Use of fourier transform ir spectroscopy for the study of saliva composition. J Appl Spectrosc 2018;85:445-51.
230. Malon RS, Sadir S, Balakrishnan M, Córcoles EP. Saliva-based biosensors: noninvasive monitoring tool for clinical diagnostics. Biomed Res Int 2014;2014:962903.
231. Dhanya M, Hegde S. Salivary glucose as a diagnostic tool in Type II diabetes mellitus: a case-control study. Niger J Clin Pract 2016;19:486-90.
232. Bihar E, Wustoni S, Pappa AM, Salama KN, Baran D, Inal S. A fully inkjet-printed disposable glucose sensor on paper. npj Flex Electron 2018;2:30.
233. Ciui B, Tertis M, Feurdean CN, et al. Cavitas electrochemical sensor toward detection of N-epsilon (carboxymethyl)lysine in oral cavity. Sens Actuators B Chem 2019;281:399-407.
234. Arakawa T, Tomoto K, Nitta H, et al. A wearable cellulose acetate-coated mouthguard biosensor for in-vivo salivary glucose measurement. Anal Chem 2020;92:12201-7.
235. Lim HR, Lee SM, Park S, et al. Smart bioelectronic pacifier for real-time continuous monitoring of salivary electrolytes. Biosens Bioelectron 2022;210:114329.
236. García-Carmona L, Martín A, Sempionatto JR, et al. Pacifier biosensor: toward noninvasive saliva biomarker monitoring. Anal Chem 2019;91:13883-91.
237. Fogh-andersen N, Altura BM, Altura BT, Siggaard-andersen O. Composition of interstitial fluid. Clin Chem 1995;41:1522-5.
238. Sun H, Zheng Y, Shi G, Haick H, Zhang M. Wearable clinic: from microneedle-based sensors to next-generation healthcare platforms. Small 2023;19:e2207539.
239. Dervisevic M, Alba M, Prieto-simon B, Voelcker NH. Skin in the diagnostics game: Wearable biosensor nano- and microsystems for medical diagnostics. Nano Today 2020;30:100828.
240. Friedel M, Thompson IAP, Kasting G, et al. Opportunities and challenges in the diagnostic utility of dermal interstitial fluid. Nat Biomed Eng 2023;7:1541-55.
241. Sharma S, Huang Z, Rogers M, Boutelle M, Cass AE. Evaluation of a minimally invasive glucose biosensor for continuous tissue monitoring. Anal Bioanal Chem 2016;408:8427-35.
242. Yang J, Gong X, Chen S, et al. Development of smartphone-controlled and microneedle-based wearable continuous glucose monitoring system for home-care diabetes management. ACS Sens 2023;8:1241-51.
243. Rao G, Glikfeld P, Guy RH. Reverse iontophoresis: development of a noninvasive approach for glucose monitoring. Pharm Res 1993;10:1751-5.
244. Yao Y, Chen J, Guo Y, et al. Integration of interstitial fluid extraction and glucose detection in one device for wearable non-invasive blood glucose sensors. Biosens Bioelectron 2021;179:113078.
245. De la Paz E, Barfidokht A, Rios S, Brown C, Chao E, Wang J. Extended noninvasive glucose monitoring in the interstitial fluid using an epidermal biosensing patch. Anal Chem 2021;93:12767-75.
246. Imani S, Bandodkar AJ, Mohan AMV, et al. A wearable chemical-electrophysiological hybrid biosensing system for real-time health and fitness monitoring. Nat Commun 2016;7:11650.
247. Hong YJ, Lee H, Kim J, et al. Multifunctional wearable system that integrates sweat-based sensing and vital-sign monitoring to estimate pre-/post-exercise glucose levels. Adv Funct Mater 2018;28:1805754.
248. Gil B, Anastasova S, Yang GZ. A smart wireless ear-worn device for cardiovascular and sweat parameter monitoring during physical exercise: design and performance results. Sensors 2019;19:1616.
249. Yu Y, Nassar J, Xu C, et al. Biofuel-powered soft electronic skin with multiplexed and wireless sensing for human-machine interfaces. Sci Robot 2020;5:eaaz7946.
250. Sempionatto JR, Lin M, Yin L, et al. An epidermal patch for the simultaneous monitoring of haemodynamic and metabolic biomarkers. Nat Biomed Eng 2021;5:737-48.
251. Sempionatto JR, Nakagawa T, Pavinatto A, et al. Eyeglasses based wireless electrolyte and metabolite sensor platform. Lab Chip 2017;17:1834-42.
252. Park J, Sempionatto JR, Kim J, et al. Microscale biosensor array based on flexible polymeric platform toward lab-on-a-needle: real-time multiparameter biomedical assays on curved needle surfaces. ACS Sens 2020;5:1363-73.
253. Yokus MA, Songkakul T, Pozdin VA, Bozkurt A, Daniele MA. Wearable multiplexed biosensor system toward continuous monitoring of metabolites. Biosens Bioelectron 2020;153:112038.
254. Misra S, Oliver NS. Utility of ketone measurement in the prevention, diagnosis and management of diabetic ketoacidosis. Diabet Med 2015;32:14-23.
255. Forrow NJ, Sanghera GS, Walters SJ, Watkin JL. Development of a commercial amperometric biosensor electrode for the ketone D-3-hydroxybutyrate. Biosens Bioelectron 2005;20:1617-25.
256. Wang CC, Hennek JW, Ainla A, et al. A paper-based “pop-up” electrochemical device for analysis of beta-hydroxybutyrate. Anal Chem 2016;88:6326-33.
257. Teymourian H, Moonla C, Tehrani F, et al. Microneedle-based detection of ketone bodies along with glucose and lactate: toward real-time continuous interstitial fluid monitoring of diabetic ketosis and ketoacidosis. Anal Chem 2020;92:2291-300.
258. Moon JM, Del Caño R, Moonla C, et al. Self-testing of ketone bodies, along with glucose, using touch-based sweat analysis. ACS Sens 2022;7:3973-81.
259. Vargas E, Teymourian H, Tehrani F, et al. Enzymatic/immunoassay dual-biomarker sensing chip: towards decentralized insulin/glucose detection. Angew Chem Int Ed Engl 2019;58:6376-9.
260. Liu S, Shen Z, Deng L, Liu G. Smartphone assisted portable biochip for non-invasive simultaneous monitoring of glucose and insulin towards precise diagnosis of prediabetes/diabetes. Biosens Bioelectron 2022;209:114251.
261. Buxton OM, Cain SW, O’Connor SP, et al. Adverse metabolic consequences in humans of prolonged sleep restriction combined with circadian disruption. Sci Transl Med 2012;4:129ra43.
262. Munje RD, Muthukumar S, Prasad S. Lancet-free and label-free diagnostics of glucose in sweat using Zinc Oxide based flexible bioelectronics. Sen Actuators B Chem 2017;238:482-90.
263. Tian G, Zhou Z, Li M, Li X, Xu T, Zhang X. Oriented antibody-assembled metal-organic frameworks for persistent wearable sweat cortisol detection. Anal Chem 2023;95:13250-7.
265. Kim J, Sempionatto JR, Imani S, et al. Simultaneous monitoring of sweat and interstitial fluid using a single wearable biosensor platform. Adv Sci 2018;5:1800880.
266. Tehrani F, Teymourian H, Wuerstle B, et al. An integrated wearable microneedle array for the continuous monitoring of multiple biomarkers in interstitial fluid. Nat Biomed Eng 2022;6:1214-24.
267. Wang M, Yang Y, Min J et al. A wearable electrochemical biosensor for the monitoring of metabolites and nutrients. Nat Biomed Eng 2022;6:1225-35.
268. Rodríguez-Rodríguez I, Rodríguez JV, Chatzigiannakis I, Zamora Izquierdo MÁ. On the possibility of predicting glycaemia ‘on the fly’ with constrained iot devices in type 1 diabetes mellitus patients. Sensors 2019;19:4538.
269. Sudharsan B, Peeples M, Shomali M. Hypoglycemia prediction using machine learning models for patients with type 2 diabetes. J Diabetes Sci Technol 2015;9:86-90.
270. Reifman J, Rajaraman S, Gribok A, Ward WK. Predictive monitoring for improved management of glucose levels. J Diabetes Sci Technol 2007;1:478-86.
271. Li K, Liu C, Zhu T, Herrero P, Georgiou P. GluNet: a deep learning framework for accurate glucose forecasting. IEEE J Biomed Health Inform 2020;24:414-23.
272. Gu W, Zhou Y, Zhou Z, et al. SugarMate: non-intrusive blood glucose monitoring with smartphones. Proc ACM Interact Mob Wearable Ubiquitous Technol 2017;1:1-27.
273. Beauchamp J, Bunescu R, Marling C, Li Z, Liu C. LSTMs and deep residual networks for carbohydrate and bolus recommendations in type 1 diabetes management. Sensors 2021;21:3303.
274. Li K, Daniels J, Liu C, Herrero P, Georgiou P. Convolutional recurrent neural networks for glucose prediction. IEEE J Biomed Health Inform 2020;24:603-13.
275. Zhu T, Li K, Kuang L, Herrero P, Georgiou P. An insulin bolus advisor for type 1 diabetes using deep reinforcement learning. Sensors 2020;20:5058.
276. Aliberti A, Pupillo I, Terna S, et al. A multi-patient data-driven approach to blood glucose prediction. IEEE Access 2019;7:69311-25.
277. Deng Y, Lu L, Aponte L, et al. Deep transfer learning and data augmentation improve glucose levels prediction in type 2 diabetes patients. NPJ Digit Med 2021;4:109.
278. Bois M, El Yacoubi MA, Ammi M. Adversarial multi-source transfer learning in healthcare: application to glucose prediction for diabetic people. Comput Methods Programs Biomed 2021;199:105874.
279. Sankhala D, Sardesai AU, Pali M, et al. A machine learning-based on-demand sweat glucose reporting platform. Sci Rep 2022;12:2442.
280. Bertachi A, Viñals C, Biagi L, et al. Prediction of nocturnal hypoglycemia in adults with type 1 diabetes under multiple daily injections using continuous glucose monitoring and physical activity monitor. Sensors 2020;20:1705.
281. Plis K, Bunescu R, Marling C, Shubrook J, Schwartz F. A machine learning approach to predicting blood glucose levels for diabetes management. In: Workshops at the Twenty-Eighth AAAI conference on artificial intelligence. 2014. Available from: http://smarthealth.cs.ohio.edu/pubs/AAAI-WS-2014.pdf. [Last accessed on 15 Apr 2024]
282. Yang J, Li L, Shi Y, Xie X. An ARIMA model with adaptive orders for predicting blood glucose concentrations and hypoglycemia. IEEE J Biomed Health Inform 2019;23:1251-60.
283. Parrilla M, Detamornrat U, Domínguez-Robles J, Tunca S, Donnelly RF, De Wael K. Wearable microneedle-based array patches for continuous electrochemical monitoring and drug delivery: toward a closed-loop system for methotrexate treatment. ACS Sens 2023;8:4161-70.
284. Teymourian H, Parrilla M, Sempionatto JR, et al. Wearable electrochemical sensors for the monitoring and screening of drugs. ACS Sens 2020;5:2679-700.
Cite This Article
Export citation file: BibTeX | RIS
OAE Style
Jung HH, Lee H, Yea J, Jang KI. Wearable electrochemical sensors for real-time monitoring in diabetes mellitus and associated complications. Soft Sci 2024;4:15. http://dx.doi.org/10.20517/ss.2024.02
AMA Style
Jung HH, Lee H, Yea J, Jang KI. Wearable electrochemical sensors for real-time monitoring in diabetes mellitus and associated complications. Soft Science. 2024; 4(2): 15. http://dx.doi.org/10.20517/ss.2024.02
Chicago/Turabian Style
Jung, Han Hee, Hyeokjun Lee, Junwoo Yea, Kyung-In Jang. 2024. "Wearable electrochemical sensors for real-time monitoring in diabetes mellitus and associated complications" Soft Science. 4, no.2: 15. http://dx.doi.org/10.20517/ss.2024.02
ACS Style
Jung, HH.; Lee H.; Yea J.; Jang K.I. Wearable electrochemical sensors for real-time monitoring in diabetes mellitus and associated complications. Soft. Sci. 2024, 4, 15. http://dx.doi.org/10.20517/ss.2024.02
About This Article
Copyright
Data & Comments
Data
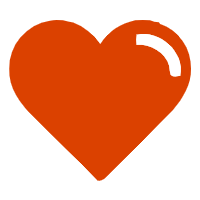

Comments
Comments must be written in English. Spam, offensive content, impersonation, and private information will not be permitted. If any comment is reported and identified as inappropriate content by OAE staff, the comment will be removed without notice. If you have any queries or need any help, please contact us at support@oaepublish.com.